Summary
Databases and analytics architectures have gone through several generational shifts. A substantial amount of the data that is being managed in these systems is related to customers and their interactions with an organization. In this episode Tasso Argyros, CEO of ActionIQ, gives a summary of the major epochs in database technologies and how he is applying the capabilities of cloud data warehouses to the challenge of building more comprehensive experiences for end-users through a modern customer data platform (CDP).
Announcements
- Hello and welcome to the Data Engineering Podcast, the show about modern data management
- Data lakes are notoriously complex. For data engineers who battle to build and scale high quality data workflows on the data lake, Starburst powers petabyte-scale SQL analytics fast, at a fraction of the cost of traditional methods, so that you can meet all your data needs ranging from AI to data applications to complete analytics. Trusted by teams of all sizes, including Comcast and Doordash, Starburst is a data lake analytics platform that delivers the adaptability and flexibility a lakehouse ecosystem promises. And Starburst does all of this on an open architecture with first-class support for Apache Iceberg, Delta Lake and Hudi, so you always maintain ownership of your data. Want to see Starburst in action? Go to dataengineeringpodcast.com/starburst and get $500 in credits to try Starburst Galaxy today, the easiest and fastest way to get started using Trino.
- Data projects are notoriously complex. With multiple stakeholders to manage across varying backgrounds and toolchains even simple reports can become unwieldy to maintain. Miro is your single pane of glass where everyone can discover, track, and collaborate on your organization's data. I especially like the ability to combine your technical diagrams with data documentation and dependency mapping, allowing your data engineers and data consumers to communicate seamlessly about your projects. Find simplicity in your most complex projects with Miro. Your first three Miro boards are free when you sign up today at dataengineeringpodcast.com/miro. That’s three free boards at dataengineeringpodcast.com/miro.
- Your host is Tobias Macey and today I'm interviewing Tasso Argyros about the role of a customer data platform in the context of the modern data stack
Interview
- Introduction
- How did you get involved in the area of data management?
- Can you describe what the role of the CDP is in the context of a businesses data ecosystem?
- What are the core technical challenges associated with building and maintaining a CDP?
- What are the organizational/business factors that contribute to the complexity of these systems?
- The early days of CDPs came with the promise of "Customer 360". Can you unpack that concept and how it has changed over the past ~5 years?
- Recent years have seen the adoption of reverse ETL, cloud data warehouses, and sophisticated product analytics suites. How has that changed the architectural approach to CDPs?
- How have the architectural shifts changed the ways that organizations interact with their customer data?
- How have the responsibilities shifted across different roles?
- What are the governance policy and enforcement challenges that are added with the expansion of access and responsibility?
- What are the most interesting, innovative, or unexpected ways that you have seen CDPs built/used?
- What are the most interesting, unexpected, or challenging lessons that you have learned while working on CDPs?
- When is a CDP the wrong choice?
- What do you have planned for the future of ActionIQ?
Contact Info
Parting Question
- From your perspective, what is the biggest gap in the tooling or technology for data management today?
Closing Announcements
- Thank you for listening! Don't forget to check out our other shows. Podcast.__init__ covers the Python language, its community, and the innovative ways it is being used. The Machine Learning Podcast helps you go from idea to production with machine learning.
- Visit the site to subscribe to the show, sign up for the mailing list, and read the show notes.
- If you've learned something or tried out a project from the show then tell us about it! Email hosts@dataengineeringpodcast.com) with your story.
- To help other people find the show please leave a review on Apple Podcasts and tell your friends and co-workers
Links
- Action IQ
- Aster Data
- Teradata
- Filemaker
- Hadoop
- NoSQL
- Hive
- Informix
- Parquet
- Snowflake
- Spark
- Redshift
- Unity Catalog
- Customer Data Platform
- CDP Market Guide
- Kaizen
The intro and outro music is from The Hug by The Freak Fandango Orchestra / CC BY-SA
Sponsored By:
- Starburst: 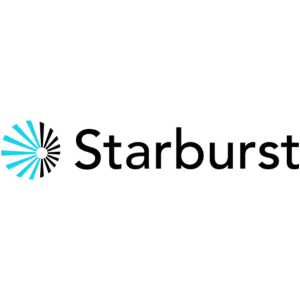 This episode is brought to you by Starburst - a data lake analytics platform for data engineers who are battling to build and scale high quality data pipelines on the data lake. Powered by Trino, Starburst runs petabyte-scale SQL analytics fast at a fraction of the cost of traditional methods, helping you meet all your data needs ranging from AI/ML workloads to data applications to complete analytics. Trusted by the teams at Comcast and Doordash, Starburst delivers the adaptability and flexibility a lakehouse ecosystem promises, while providing a single point of access for your data and all your data governance allowing you to discover, transform, govern, and secure all in one place. Starburst does all of this on an open architecture with first-class support for Apache Iceberg, Delta Lake and Hudi, so you always maintain ownership of your data. Want to see Starburst in action? Try Starburst Galaxy today, the easiest and fastest way to get started using Trino, and get $500 of credits free. [dataengineeringpodcast.com/starburst](https://www.dataengineeringpodcast.com/starburst)
- Miro: 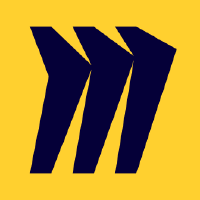 Data projects are notoriously complex. With multiple stakeholders to manage across varying backgrounds and toolchains even simple reports can become unwieldy to maintain. Miro is your single pane of glass where everyone can discover, track, and collaborate on your organization's data. I especially like the ability to combine your technical diagrams with data documentation and dependency mapping, allowing your data engineers and data consumers to communicate seamlessly about your projects. Find simplicity in your most complex projects with Miro. Your first three Miro boards are free when you sign up today at [dataengineeringpodcast.com/miro](https://www.dataengineeringpodcast.com/miro).
Hello, and welcome to the Data Engineering podcast, the show about modern data management. Data lakes are notoriously complex. For data engineers who battle to build and scale high quality data workflows on the data lake, Starburst powers petabyte scale SQL analytics fast at a fraction of the cost of traditional methods so that you can meet all of your data needs ranging from AI to data applications to complete analytics. Trusted by teams of all sizes, including Comcast and DoorDash, Starburst is a data lake analytics platform that delivers the adaptability and flexibility a lakehouse ecosystem promises. And Starburst does all of this on an open architecture with first class support for Apache Iceberg, Delta Lake and Hoody, so you always maintain ownership of your data.
Want to see Starburst in action? Go to dataengineeringpodcast.com/starburst and and get $500 in credits to try Starburst Galaxy today, the easiest and fastest way to get started using Trino. Data projects are notoriously complex With multiple stakeholders to manage across varying backgrounds and tool chains, even simple reports can become unwieldy to maintain. Miro is your single pane of glass where everyone can discover, track, and collaborate on your organization's data. I especially like the ability to combine your technical diagrams with data documentation and dependency mapping, allowing your data engineers and data consumers to communicate seamlessly about your projects. Find simplicity in your most complex projects with Miro.
Your first 3 Miro boards are free when you sign up today at data engineering podcast dotcom/miro. That's 3 free boards at data engineering podcast.com/mir0. Your host is Tobias Macey, and today I'm interviewing Tassos Agueros about the role of a customer data platform in the context of the modern data stack. So, Tassos, can you start by introducing yourself?
[00:02:03] Unknown:
Yes. Thank you for having me here. So I'm the founder and CEO of a company called Action AQ. We're 1 of the leading CDPs, compostable CDPs as as we call it, and we can talk about what that is and why it's important. In terms of quick background, I grew up in Greece. I, came to the US to do a PhD in computer science at Stanford. I had done a lot of data mining and databases, academically. And so I started working on distributed database systems, large scale database system at Stanford. And shortly after that, I dropped out and just started 1 of the first what used to be called big data database companies in the mid 2000.
That company was called Aster Data. And, you know, it was 1 of the first databases that could really scale to, you know, tens of terabytes of data at relatively low cost. It was all based on commodity hardware. And I sold that to Teradata in 2011. You know, we had a good exit, and Teradata, at the time, and to some extent, still is 1 of the main database providers, to the largest enterprises in the world. I spent 3 years there. That was a great time and very educational. I learned the ins and outs of, database, technology and business.
And then, you know, I wanted to build something that had, data at its core, but was more focused on the business user. Because the biggest gap I saw from my time at Teradata was, how do you bridge the database systems and what the business had to do with that data and those database systems, and you make it more, effective. So I started ActionIQ with a vision of, empowering the business to be more autonomous, more automated, more self-service, with, customer data and do it at scale. And, actually, I think, we've had a great run. You know, we've been around for 7, 8 years now. We have, some of the biggest enterprises.
We're proud to call our partners and our customers. And, we were 1 of the very first CDP's customer data platforms, and it's been fascinating to be part of that company, category creation and category evolution.
[00:04:17] Unknown:
So that's the the story, and then that's all. And do you remember how you first got started working in data?
[00:04:23] Unknown:
When I first started working in data, I I think I think I was about 11 years old. And what I was trying to do is my we we lived in a in a mid rise building. And in Greece, usually, the way it works is that 1 of the tenants of the building, they rotate, becomes kind of the administrator to manage all the expenses of the building and the payments, the expenses that runs the building p and l. So it was kind of a rotation. And everything was done manually. Right? That was in the nineties. And so I was, my my dad had bought me a Macintosh. That's a professor of mathematics, and he came back from the US, and he brought this weird box that was an Apple Macintosh. It was an LC, model at the time.
And, you know, I wanted to build the database to automate, the building management, all the expenses and the payments and everything else, so I I discovered FileMaker, which is, an application that would run-in Mac. I think Apple bought it. And FileMaker was almost like what you would call today a low code database system. Actually, very similar to the today's low code systems, but, of course, you know, it was nineties technology. And so, I remember I worked almost, 20 hours straight, and I came up with a prototype of that. And it actually worked, you know, for the next few years. My parents used it, and I served it with others in the building that would let them use our computer and the application and built on FileMaker. So that was the first time. It was a really long time ago.
And then, you know, academically, I was always in the database field, so I've been I've been doing data my whole life more or less.
[00:06:08] Unknown:
Given the span of time that you have been working in the data space and working both academically and in industry with database systems, I'm wondering if you can give your sense of how you would categorize the major epochs of database technology from when you first started working in that space to where we are today?
[00:06:29] Unknown:
Yeah. I mean, it's been fascinating. You know, my I'd say that every 5 to 7 years, database architecture shifts. And the reason database architecture shifts is because database always say is chasing performance. Right? Database always say for there's always more data than money. And you always want more performance out of the amount of funds or money that you have. Right? So databases is a history of chasing performance. And to chase performance, you have to take advantage of the latest evolution in hardware and software and networking. And so as hardware and networking is changing, database architecture is changing. And, you know, I I think kind of where I started following, databases was in the nineties, and you had the the famous database wars with Informix fighting Oracle, essentially.
And that was all done based on you would you would have to buy very expensive hardware to run those databases at scale. Right? So that time, if you wanted to build a large scale database, you had to buy this huge closet of hard drives, then you had to to buy a multiprocessor computer from, like, IBM. We're talking about you you probably pay, like, a few millions for the disk array, and you pay a few millions for, like, a, you know, 8 CPU processing. Right? That's probably 1, 000 times lower than an iPhone. Right? Probably more than that now. And you would connect the 2, and that's how you would build, like, a 1 terabyte database system. Right? That that's what's large scale data warehousing.
And the only ones that could afford to do this in the late nineties, early 2000 was companies that whose data was very valuable. So banks. Right? If you're a bank, you know, the 1 transaction from your customer is very valuable. Right? If you're a tel co, you know, 1 household transaction is very valuable. So very expensive and also very limited. When my last company came into play in 2005, what the way I started Astra Data was, my adviser, at the time, David Sheridan at Stanford, he was the 1st investor in Google. He he gave him the 1st check, first name to investor, and he had followed Google very closely. He came to me. He said, hey. You're a database guy.
Can you build a database that uses the same architecture commodity hardware? Right? Very low cost that Google uses for search. And that was, you know, pre had to pre all of that stuff. Right? That that was when database was still using very expensive hardware. So we started this effort to have this massively parallel, like, databases. And we would buy cheaper it would still be, like, $10, 15, 000 per per server, right, but cheaper than the multiprocessor units. And we would use processing and storage together. So you have CPUs on the box and the storage in the box, and we would put the fastest interconnect we could afford, which is some gigabit Ethernet at the time.
And that's how you could build a bot as a system, right, that would have 50, 100 servers. And you could build a database that would be, like, you know, 25, 30 terabytes at 1 tenth of the cost of what it would cost you to build, you know, a 2 terabyte database the old way. Of course, there were trade offs. Right? Because the way you partition the data matter. Right? So you if you did processing where the data was already collocated, that was fast. But the interconnects at the time, the networking was not very fast. Right? So every time you had to shuffle data, it would be very slow. That being said, so many companies at the time, right, they the Internet was blowing up. So many companies had high volume, low value data, and they were desperate for a way to do some processing on the data without having to spend $10, 000, 000.
Our first customer at at Aster was Myspace, which is 1 of these companies. And it was a huge deal. Right? It was 1 of the biggest deals I've done in my life. That was the first deal I tested data. And the rest was history. Right? So that was kind of the mid to late 2000. It wasn't only us. It was, like, 2 or 3 of us doing it, then we got all got acquired in 2010 when big data blew up. But then what took over after us was Hadoop. Right? So Hadoop did had a similar architecture to what we were doing. It was a bit more developer focused. And in the beginning, the whole, I you know, had idea with Hadoop is that you didn't need SQL. Right? That was back in the NoSQL days, so everybody was talking about how SQL was dead and that, you know, you just need to write Java code. Obviously, that was wrong. And we knew it was wrong, but, you know, there was a lot of excitement around the idea at the time.
And Hadoop started as a development platform, right, where you could do MapReduce raw Java on top of raw distributed files. Right? Hadoop file system and then MapReduce on top. Very quickly, though, it became apparent that you had to have SQL. And, you know, systems like Hive came out. Right? And Hive was inspired actually to to a good extent from Aster. The after our SQL engine was called Beehive because, you know, we thought about it as kind of a, you know, conglomeration of, of these doing work together, so we called it Beehive. And at the time, we were POC'd, by Facebook.
And Facebook POC'd us there, POC'd Beyhive, and then they turned around, and they wrote Hive, which is kind of an open source SQL engine on top of HDFS. The problem with with that is that Hadoop was never designed to be a database. And this is something I'm seeing over and over again. Right? Sometimes you take a system that became very popular for some reason, then you try to make it something different, and that never works because the DNA and the vision of the people that build the system, the architecture is very different even if it sounds the same. So the way the data was stored, the way that, you know, that the tasks were managed and run and deployed, the way the SQL was managed and distributed and optimized.
It was like you tried to bolt on a SQL thing, right, on top of something very different. So Hadoop never really became the next generation data warehouse. Right? It became a data lake. Like, you could you could store a lot of data, it should be, and do some processing, some ATL maybe, but but it was never at the data warehouse. But if you take kind of the, you know, Informix, Red Brick, Oracle, right, and then Teradata as kind of generation 1 and expensive hardware, then you have the MPP databases generation 2. Right? Mostly on analytics, what I'm talking about. Then we had Hadoop kind of a generation 3. You can call it Hadoop 3.0 was MapReduce Java on top of HDFS, and Hadoop 3.5, let's call it, right, was the SQL like, retrofits on top of Hadoop.
And then you went to the latest generation, right, which is the cloud databases. And what I find fascinating with the cloud databases is that if you think about it, Informix and and, these companies had storage and processing separate. Then NPP databases like Aster and Hadoop brought it together because that was most cost effective, and then the cloud and cloud data warehouses took it apart again. Right? So we went from data and processing separate to data and processing together, and now data and processing separate. And so it sounds a bit like backtracking, but it's not. And the reason it isn't is is because we now have interconnects networking that you can have 10 gigabits non blocking at scale, which in the mid 2000, that was a dream. Right? So that, in my opinion, is the biggest thing that changed, that you can have a 1, 000 servers with storage and a 1, 000 servers with processing, and they can now exchange data.
And the network does not become the bottleneck. When I was doing, you know, our database mid 1, 000 network king was absolutely the bottleneck. And so now you have things like s 3, right, and others like it, that you can store immense amounts of data, and you can scale storage and processing independently. That is fascinating. Right? And it's fascinating we we've come to this point, and that changed the whole game. And as we were discussing earlier, when the hardware changes so drastically, the older generation of databases almost never make it to the new generation. You almost have to do a complete rewrite because the architectural assumptions change fundamentally.
So now it's a brave new world. Right? Because, you get a lot of advantages, mainly that you can separate the concerns with how you where you store and how you manage the data when it's stored and then how you process the data. You also get a little bit of elasticity. Right? You can scale up and down your processing resources to respond to queries, whether it's volume or complexity of queries. And that kind of brings us to today, I would say. And, again, I'm I'm giving a very short version. Right? And I'm not mentioning probably a lot of things I should mention, but in the interest of time, that's the best I can do as a short version of, analytics database history.
[00:16:01] Unknown:
An interesting element too of where we are now to your point, a lot of the Hadoop ecosystem was built up around the shortcomings of that core MapReduce engine and the HDFS file system. And now we've moved into an era where a lot of the data lakes and data lake house architectures are outgrowths of that original Hadoop ecosystem. I'm curious what you see as some of the fundamental architectural failings or shortcomings or some of the limitations that we are inheriting from that generation as we continue to try and build out these new capabilities and new architectural paradigms?
[00:16:42] Unknown:
Yeah. So I think, you know, interestingly enough, most of what we have inherited from Hadoop is storage storage formats. Right? And and, you know, things like Parkin and Iceberg and things like that. The engines the the the processing engines are all brand new. If you think about it, you know, Snowflake was a brand new engine. Right? To my knowledge, they they have a new significant code from Hadoop. Same with Databricks. Databricks was built essentially, you start as Spark. Right? And Spark had a much more flexible, processing model that had duped it. Right? It was it was in memory. It was iterative.
It was a much better foundation to build a database on top of than MapReduce was. That was huge batch iterations, essentially. AWS, Redshift. Right? Redshift was originally it was an acquisition of 1 of Astridata competitors. But by now, I would say they have completely rewritten it. Right? So they really not have a lot with Hadoop. So interestingly enough, what we get from the Hadoop era was the storage. Right? The the storage format and some of the the storage APIs. And that's what people call the data lake today. But when it comes to processing, you know, processing SQL, very little of the Hadoop stuff made it over. Right? Because, again, it was so architecturally different, Like, what you need to run high performance, high volume analytical SQL is so different from what Hadoop tried to do. It's essentially big batch CTL jobs that, had to be written from scratch. So yeah. So most of the database systems, I would say, are are full rewrites or or brand new or different, at least.
And then the Hadoop stuff, you know, we kept the storage, but I think the rest doesn't have, a great future at this point.
[00:18:27] Unknown:
I think another interesting lesson that came out of the Hadoop era was the conceptual approach to big data and data acquisition and storage where in the early to mid phase of the Hadoop era, it was just throw all the data in there. Eventually, it'll be useful. We can do magical data science, and everything will be amazing. And then through a lot of failures and hard won lessons, we came to the realization that actually, 1, it's not gonna magically work. 2, it's really expensive. And 3, it's actually going to put us into a lot of compliance risk and regulatory risk. And so now we are in a phase where people are trying to figure out how to be more judicious and targeted with the data that we acquire and store, and it also brings along a much higher burden of governance if you have all that extra data lying around.
And I'm wondering what you see as the, next generation of database technologies or database capabilities that we either are building towards or should be thinking of as we continue to evolve our understanding and capabilities around data systems.
[00:19:40] Unknown:
That's exactly right. Yeah. It's a great point. And, you know, the reason why that worked, what you say, you know, just dump the data now, think about it later. The reason why it worked in the early days of Hadoop, in my opinion, there were 2 things. 1, the data engineer and the data analyst was the same person. The person that would load the data and process the data and analyze the data was the same person. If that's the case, that kind of makes more sense. Right? Because if you love the data, you know the data. Right? You can then go back and do whatever you want. And you don't quite know how you're gonna use it, so you might as well do not do premature optimization.
Right? That was the argument. But but that only works in an experimentation context or in a very small organization. Right? In a big enterprise organization, the people that build the data pipelines and the analysts are 2 different personas, so their requirements are very different. The second reason it it worked, I think, is that early days, Hadoop was not production critical systems. Hadoop was a, you know, it was a sandbox, right, where you can throw some data and do some cool stuff. And, oh my god, it was so much faster than having to go through the, you know, red tape to go through the warehouse or whatever. Right? And people were just it was a a a a breath of fresh air, right, in some ways.
However, what happens with all these systems, and it's always the same. Right? You start with an experimentation box. You do something interesting. That interesting thing has value for the business. You take it a bit further, not break. It becomes a production workflow. Right? A workload. And the moment it becomes a production workload, you know, and the enterprise comes and puts a lot of red tape around it. Right? So what starts as fun and games eventually becomes, a mission critical production workflow, and then you can't experiment anymore. To your question, though, I think modern database systems have to provide flexibility. There's still value in dumping data in the data lake without doing much more processing.
Because a lot of your data is new, you don't know what to do with it yet and all that stuff. But when you're in a enterprise environment, the mission critical workloads and mission critical data, it has to have quality guarantees, schema guarantees. Right? All that stuff. And, you know, there's new language these days. Right? People talk about bronze and silver and golden tables and records. Right? And they use this terminology to explain the progression from low value, unstructured, low quality data to high value, highly structured, high quality data. I don't think we can go back, though, to the era where everything had to be perfectly structured.
At the same time, this idea that you don't need to structure anything, you just dump everything in the data lake, and you don't worry about it. It doesn't work in an enterprise environment. But flexibility, I think, is the name of the game, and I think a lot of the modern database technologies are competing to say, hey. You have many workflows. These different workflows have different requirements for quality and structure, and we want to support all of it and also give you a lot of observability so that you know what's going on and how to fix things.
[00:22:44] Unknown:
And I think it's interesting to think about too how the current generation of data systems are building an increased reliance on the overarching metadata platform to be able to get visibility of data across an enterprise or even across a particular product scope because of the different systems involved and being able to view things like lineage. And you were talking earlier about data quality enforcement and validation. And I'm interested to see how those capabilities start to be incorporated into the database engine itself or maybe the metadata platform becomes the sys like, the organization wide database with federating to those underlying actual compute capabilities.
[00:23:27] Unknown:
Yeah. I I think, you know, this is, that that's something interesting very interesting in my mind because, you know, in the past, databases, you know, that that that had you could get a list of tables. Right? But that's about the everything else was custom code around it. And now you see, you know, folks like date Databricks. Right? They have Unity catalog, which is it's a full metadata system, right, that's that's that is trying to cover not just what's in Databricks, but what's across the whole enterprise. And so I think you see modern database companies going beyond databases. Right? Not only did they provide a SQL database engine, but they provide they're almost like data management companies.
And they're expected to provide tooling that will help Nasus with their SQL queries executing them, you know, with good price performance, but they expect to help with the full end to end life cycle management of data, which is also why you see, you know, Databricks and Snowflake and others, AWS, right, expanding to machine learning and model building, which which, you know, like, 10 years ago, that was a completely different category of software. But because everybody's using the same data for everything now, you know, they they feel like they have to provide an ML development environment as well as a database environment as well as all the metadata as well as all the data lake storage and the ATL tooling. Right? So it's almost like you get, like, you know, an old school Informatica, right, and SAS, maybe a Teradata.
Now it's all 1 company. Right? And that's how companies compete to say, we helping build models. We can help you manage all your data even outside of the database. We can help you with storage. We can help you with processing. And I think, you know, they're gonna keep expanding and expanding and expanding. Right? Just like, you know, Microsoft in the old days started being an operating system, and then they built operate, you know, office applications, and then they built enterprise applications and everything else. It's actually a very exciting time to be a database company just because because of the cloud mostly, right, and how flexible and accessible it is.
The opportunity has is much more broad now than what it was as a database company 20 years ago.
[00:25:49] Unknown:
Databases are interesting at least for people who are very deep in the weeds technically because of all of the that's involved in building these systems, but they're effectively useless unless you're doing something interesting with the data that they hold. And now in your current role, you're working very closely in this space of customer data platforms or CDPs. And I'm wondering if you can give a bit of an overview about the role of the CDP in the context of the business data ecosystem. I've done other episodes focused on what are CDPs and discussing a little bit about the idea of composable CDPs. So I'll add links in the show notes to some of those folks who wanna dig a bit deeper there. But for the purpose of the business, what role does that CDP play?
[00:26:35] Unknown:
Yeah. And and maybe it would help if I it's a very short story about how why even got into the CDP space. Right? Because I think that answers part of the question. But, you know, when I was at Aster and then at Teradata, you know, we work with 100 of of Global 2, 000 Enterprises. And my observation I had 2 observations. 1, most of the data in this enterprise data warehouse was customer data specifically. I mean, a database is supposed to manage any type of data. Right? So it really has a long tail of what kind of data you manage, right, and what you do with it. But if you look at customer data, I would argue that if you take companies like Snowflake or Databricks, 80% of their market cap is because of customer data or customer data related use Right? It's it's a huge majority, in my opinion.
So that's 1 big realization I had. And the second realization was that what the amount of customer data you had in the data lake today, right, or the data warehouse was about a 1000 times more than what would end up being leveraged in a business application. Right? If you take something very simple, like, you know, you have an application that sends out email. Right? Like, you know, like, in, you know, 1 of the marketing clouds. Right? Like, Salesforce or in Adobe. Right? The amount of data you have in these platforms, the amount of customer that you have in this platform is, like, maybe 1% of the customer data available in the data warehouse.
So, you know, in the context of this is, you know, you you you enterprise has spent, you know, tens of 1, 000, 000 of dollars, right, maybe more to build this very large scale data lake lakehouse enterprise warehouse. You get so much customer data there. And then what? Right? Then what happens with that data? I mean, obviously, if you're an engineer, you know, SQL can go in, get some insights, but most of the people that drive the customer experience, that drive decisions based on, you know, customer insights, they're not SQL engineers. Right? They're not engineers. What do you do with that?
And so CDP, the way I think about it is from a stack if you think about it, the the data stack, right, it it sits on top of the data warehouse, right, and below the marketing applications or the customer applications in general. Today, this goes way beyond marketing. Right? It can be call center. It can be operations. It can be all kinds of things. And so from a from that perspective, CDP is almost at the same level as BI. Right? If you think about BI, it also sits on top of the day warehouse, and it's supposed to be a self-service automation tool for business to get insights.
CDP is similar, but it's not so much about providing aggregate insights on customers, which is what a BI tool would do. It's all about how do we drive the right action for our customers, orchestrated across all the channels, right, that we use. And so there's sometimes people use that term data activation, right, to describe the CDP, and, I mean, I think that's that's reasonable. Data people, by the way, use the term activation very different from business people. When a business person says activation, it means, like, a campaign, like an ad campaign.
When a data person says activation, it means essentially getting the data and leveraging the data with some, customer, facing application. But that's kind of where it is in the stack. So the term CDP is a bit of an misnomer. Right? Because customer data platform and and for many years, less today, I think, for many years, there was confusion because someone, you know, some data engineer or IT person would hear CDP, and they would think like, oh, that's a, you know, that's a, like, data lake with customer data. Right? Or that's a customer 360. But that is not true. Right? CDP is 1 layer above that. Right? The the goal of the CDP is not to create a custom customer 360, although some CDPs try to do that. But in my opinion, that's outdated.
Why is it outdated? Because of everything we spoke about before, today, you have these super powerful data management systems, right, that can store unlimited amounts of data and do all kinds of structure and queries on top of it. And you want all your customer data in 1 place integrated with the rest of the enterprise data. So you don't necessarily want to take some of your customer data and put it in a proprietary application that sits out to the side, right, which is what some CDPs would argue you want to do. I call those the data integration CDPs. Right? Some vendors do that. Try to say, we will take your customer data, integrate it, connect it, and then we will store your customer data, and then you can access the customer data in our own proprietary engine.
But I feel that goes against the tide of the data lake and the lakehouse and how you wanna have all your customer data in 1 place in the cloud in open format integrated with the rest of your enterprise data. And so the whole data integration CDP concept in my mind is a bit of a historical error. And, by the way, for those that interested, we we have a CDP market guide where we talk specifically about vendors and who's what and what they're doing. You can people can download that from the actionIQ.com website if they're interested. But what ActionIQ does, we're we're not trying to integrate all the customer data in our own proprietary thing. We sit on top of a Databricks or a Snowflake or a Teradata, and we provide this extremely powerful user interface that automates a lot of the CX operations, allows the business users without writing any SQL to do their own hyper targeting, do their own orchestration, create customer journeys, and then activate them through different applications, different channels. Right?
So that's what what CDPs in my mind. So I call that, orchestration or data activation CDP. And then there's another category of CDPs, which is x tag managers. Right? They're folks that started as tag managers, and then they tried to pivot to become xDPs. And just like Hadoop couldn't become a SQL database, even though that ended up being the right thing to do because we're so different, The tag management CDPs, they're 90% tag management and 10% CDPs today. Right? It's completely different architecture DNA vision. Right?
And then we see the same playing out a little bit with the reverse ideal guys. Right? Reverse ideal is a it's it's an interesting way to do ATL. Right? Move data from the data warehouse to the applications. But just like Hadoop tried to become a SQL database and just like the tag manager folks tried to become CDPs, It's very hard to take an ETL tool and make it kind of a business focus, you know, data activation orchestration CDP. Right? But, you know, CDP has a history of vendors that start at something, then they don't like what they are, then they're looking for a bigger, you know, more ambitious vision that I call as become a CDP. Right?
And they try to to pivot into that category. But, you know, so far, all the sappers have failed because, you know, taking 1 product and trying to make it something very different, right, mid flight tends to be an extremely, extremely difficult thing to do. You mentioned some of the different categories of CDPs, some of the evolution that they've gone through, and how
[00:34:11] Unknown:
the different originating visions have all started to converge along this idea of the composable CDP, and people are starting from those different locations. I'm wondering too how those different architectural capabilities of where we are today and when from when people first started trying to adopt this idea or this vision of a CDP, how that has changed the conception of the responsibilities within the organization of who is supposed to be dealing with the data at what phase.
[00:34:46] Unknown:
Yeah. It's a it's a great it's a great question. Yeah. And and to clarify, so composable, CDP, what it really means at the end of the day, right, is that you can use the data warehouse for all storage and processing. And and not all the CDPs are doing that. So I would say the data integration database, they don't do it, and they will not do it because it goes against their business model. Right? The whole business model is integrating data. So data integration happens in the data warehouse. They have no business, right, more or less. So they're kind of antithetical to that. And then you have the tech manager, ex tech management CDPs, who also their focus is in capturing web data and and mobile data, and they also have, you know, very little to do with the with the data warehouse.
You have the reverse CL folks that claim to be composable CDPs. Right? But there's still reverse CL. I mean, not not just changed. Right? And then you have ActionIQ, which I think from the traditional CDPs, the orchestration CDPs were the only ones that made a full shift to composable. Meaning, today, you can deploy ActionIQ 100% on top of, you know, a Databricks or a Snowflake or a Teradata and we don't have to store any data. Right? So not everybody made the shift to composable. I would say 80% of the vendors did not, But I believe composable is where the future is.
Now with every technology, the question is, are organizations ready for composable CDP? And that goes to your question because the assumption behind composable CDP is that all your data is neatly formatted and available in 1 single place. And for most large enterprise organizations, that's not the case. That's where they're going. So for future proofing, it's very important you have the capability, but that's not where they are today, which is why what we deploy a lot of times is a hybrid model where we can have 6 to 70% of the data in, you know, a data lake or a cloud warehouse or 2. Right? We do federation essentially behind the scenes.
But then some other data still needs to be integrated and connected, and we do this the traditional way. And over time, more and more of the data lives in the data lake and less and less of the data lives in Action IQ. But there's a difference between, you know, kind of vision and reality. Right? That's why composable CDP is what everybody wants to buy, but the ability to have a hybrid model is critical to to customer success. To your point also, we see that the buyer of the CDP is changing now. It used to be that the buyer of the CDP was almost exclusively marketing, right, or business in general.
But now more and more, the chief data officer, chief technology officer, right, chief information officer, these, are the functions that take the lead because they see the CDP as a natural extension of the data management stack. Of course, business has always evolved, right, because the use cases and the value comes from there. But, you know, just like BI started kind of as a business tool, right, and it became more of a hybrid thing, right, sometimes IT buys BI, sometimes the business buys BI solution. The same thing is happening with CDP today. And in some ways, for me, personally, that's almost funny because I was selling to the chief data officer, right, with Astra Data and Teradata for almost 10 years. Right? That was my world. And then I was like, let me do something fun. I'm I'm gonna build a solution for the business.
And now I'm back to interacting and selling to, you know, the technology side of the business, which for me is great because that's my roots. That's what I did. Right? And and that's also very unique because most ADP solutions, if you look at them, right, it's it's business founders. Right? It's application folks that that thought of these applications, but the data part was an afterthought. But, you know, we're we're true database people that wanted to take a break from databases, but now we're back in the data management land. And, I just find it, you know, very, pleasantly ironic, how we are talking full circle.
[00:38:55] Unknown:
Another interesting aspect of this expansion of access, expansion of responsibility is the question of how that impacts the practical and principled elements of governance around that data and how much of the governance enforcement needs to live in the CDP environment versus how much of it is policy based and enforced from an external perspective.
[00:39:21] Unknown:
That's that's right. Yes. And there, I think, you know, we're having this conversation often. Right? Like, first of all, who owns the definitions of the data? Right? And where do these definitions live? This is not a CDP specific problem. Every time you have an analytical system that does a specific job, you have to ask yourself, is the data model and the governance for this lives in the centralized location, or does it live in the departmental location, right, or in the more application specific location? And my rule of thumb usually is that if you're using definitions in the CDP that you need beyond the CDP, Right? You it's more enterprise wide definitions. Those should absolutely live in the, you know, data warehouse, right, in the the cloud data warehouse, enterprise data warehouse.
If things are more specific to the CDP, you trade, right, some centralization for agility, and that's okay to live in the CDP. Even if the data lives in the data warehouse, by the way, the definition can live in CDP. Because if you look at Action IQ, we allow you to define essentially attributes and, you know, custom definitions from within Action IQ that are executed in the data warehouse. So I don't think it's like a black or white to say, hey. Everything should be in CDP because that's the most agile model. It's through its agile, but then you lose some governance. You have quality, consistency issues. And then if you say everything has to be centrally managed, you take a lot of autonomy away, right, from the users of the CTV.
There has to be a line, and that line depends on, you know, how the rest of the data management work, how governance works, how the organization's divided. And then for the different data, what's an enterprise wide definition or workflow, and what's more of a CDP specific definition. From a product perspective, with Action IQ, all of that is configurable. So you can implement the both of the extremes if you want or something in between, and it's really a deployment. It's a policy decision that we usually live up to the customer, to decide for themselves.
[00:41:30] Unknown:
Another interesting challenge of CDPs as they exist now versus when they were first starting to be adopted is, as you mentioned, it's no longer just marketing, who cares about it, or just sales. It has expanded to include roles like business operations, customer service, etcetera, which brings a lot of different perspectives on what information is useful, what it means for somebody to be a customer, and I'm curious how those additional perspectives have added complexity both technically and organizationally to the implementation and adoption of these systems.
[00:42:10] Unknown:
Yes. It's a great point because listen. At the end of the day, the vision of the CDP is to connect every single customer touchpoint together. And the reason for that is that doesn't matter how big of an enterprise you are. Right? You you may be, like, you know, at Fortune 50. You may have 20 departments, right, that touch the customer. You're still talking to the same person, and that person still perceives you as a single brand, a single entity, and expects consistency. Right? That's what drives great customer experience. Right? Knowledge about your customers and consistency across their different experiences.
So you may have, you know, stores. You may have call centers. You may have email. You have made direct mail. You may have, you know, field operations. Right? If you're an airline, when a customer walks up to the booth to to check-in or or do something with their flight, that's a customer that's a channel. Right? That's a customer interaction. It has to be orchestrated with everything else that the customer is receiving. If the customer just missed their flight, you don't want to try to upgrade them to business class 8, right, on the next 1. Probably not the best time, to do that. And so part of it, I think, is first of all, has nothing to do with the CDP. Right? Because if you think about it, how are the enterprises organized?
Historically, they've been organized by channel. You had an email team. You had an advertising team. Right? You you had an operations team. You had the field team. You have a customer service team. You have a call center team. And in my mind, that's where it starts. Right? That how do your organizationally structure your teams that there's more visibility? So, for example, right, instead of organizing by channel, I've seen some enterprises try to organize by life cycle. Right? Like, you try to acquire a customer, everything's 1 place. If you're in trying to get the customer more engaged, everything's 1 place. Loyalty. Right? You may have a loyalty team, and that loyalty team has access to all the channels to do what they need to do with a particular customer, and they own that particular customer in all their interactions.
You see more enterprise have a chief customer officer. Historically, the reason why marketing box CDPs is that they had great ROI use cases. Right? You can make a lot of money by buying a CDP and deploying it very quickly. But, also, the the kind of a Zoom that your customer ops your role because they own many of the customer channels. But you can make this broader and more explicit, right, by giving the CMO or maybe someone else more explicit chief customer officer responsibilities. So none of that has to do with technology per se, and you don't have all of that in place to get value from the CDP. But the more of that you the more you think about organizational structure around the single customer view, the the better it is, in my opinion.
And then beyond that, you know, nobody has a perfect structure today. Right? So what happens is you deploy CDP and you start with some use cases in some departments. The focus, especially these days, is to drive very quick ROI. Right? Get, like, a 10 x of what you paid for the CDP within the 1st year. And then from that point on, the deployment never finishes. Right? It keeps expanding and expanding and expanding because there's always more and more and more channels that you haven't covered. There's more data. There's more teams, more use cases.
And so it becomes more of a iterative approach, right, like a kaizen where you keep improving what you do with SAP every single day versus you try to boil the ocean and cover every single customer data source, every single customer channel from day 1.
[00:45:49] Unknown:
In your experience of working in this space, both from the perspective of working in database systems through the evolution that they've gone through and in your current exposure to customer data platforms? What are some of the most interesting or innovative or unexpected ways that you've seen these architectural approaches to data management, whether it's for database systems or customer data platforms?
[00:46:14] Unknown:
From a use case perspective or from an architecture perspective or technology perspective?
[00:46:20] Unknown:
Whichever 1 do you think is most interesting.
[00:46:23] Unknown:
I mean, there's so many things to talk about. Let me maybe start from the use cases. The thing that the thing that I find most fascinating with use cases, I'll say a couple of things. First of all, when you take, data from 2 completely different channels that were never talking before, you bring it together and you influence both their activations. Right? Both their what they do. You know, you take what happens inside the retail store and what direct mail goes to a customer. Right? That is the kind of stuff that's you know, brings joy and surprise to customers, right, when it happens, like, taking the clienteling and taking something very old school and bringing together or taking the example I gave. Right? What happened between an interaction with an airline agent and the email campaigns that marketing is sending out. Right?
Historically, that stuff has been completely separated, but it hits the same customer almost at the same time, and it really shouldn't be separated. So this true omnichannel orchestration is is something that is still relatively new, right, and and so much value to be gained there. Another thing that that I was very surprised was, digital ads and acquisition. When I started Action IQ, I thought we would only deal with known data post acquisition journey. Right? I would say, okay. Once you get someone acquired, you know who they are. That's we're gonna manage the rest of the life cycle from there.
But 2 or 3 years ago, everything changed with the death of the cookie. Right? So suddenly, you didn't have these funny things, right, the cookies. And even anonymous data became first party data. Right? So if you think about it, everything you know, the the modern way of doing acquisition now, post the death of the cookie, is when someone comes to your website, maybe they're anonymous, maybe you don't know them, it's still your own data. It's still first party data. It's still there. You have to capture, manage, activate with your partners. Right? It could be, like, Google or TikTok.
It's a 100% SDP use case. Right? There's some things we had to evolve, and we we put a lot of development effort into that the last 2 to 3 years into having this real time communication with the World Gardens and different ways of, connecting data together. But it allows it it's a revolutionary approach, right, because it allows brands to own their own data, own their own fate, they own their own measurement. Right? Because, frankly, cookies, right, it was always a bit of a snake oil. Right? When someone sold you a list of cookies, like, hey. These are all people that want to buy a car. Right?
Who knows? Right? Maybe the word maybe the word you had no idea of knowing, and then you still relied on third parties for measurement. So I think the in in diesel advertising, it allows brands to take control of their own data and their own pay in a way that was not possible before. And I find this revolutionary. And to be honest, it was unexpected for me. And if it wasn't for the death of the cookie, maybe, you know, we would still be in the world of DMPs and these old technologies that never really worked very well for for brands.
[00:49:30] Unknown:
And in your experience of working in this space and in particular your investment in customer data platforms? What are the most interesting or unexpected or challenging lessons that you've learned?
[00:49:41] Unknown:
Yeah. I think it's always been first of all, the confusion around the CDP space was something I wasn't expecting. Right? And and, you know, I've been in you know, with with Astra Data. We were part of the big data space. Right? It was also it was a very competitive space, and we were 1 of the 2, 3 companies, you know, that had a great exit in the end. So we did very well. But, CDP, what I I I underestimated is that I think the term itself gave license to any vendor that had did anything with data to call themselves a CDP. Right? That's how you had the tag management guys call themselves CDP, right, and getting away with it for a long time. That's how you have folks calling themselves as a DP today. Right? That's it's it's very fascinating to me.
And, also so, you know, it took a while, I feel, for the category. Like, if you look at 2024, I think this is the year where you're gonna get a lot of clarity in the city space. I think you'll see major analysts, you know, making moves, right, and and putting their positions out, which hasn't happened before. I think you'll see the market getting a lot more educated very quickly. And I think we're gonna see tremendous growth in the category overall. But the confusion around CDP and how easily vendors almost overnight, Right? And, we'll go from being a tech manager to being a CDP, like, segment, right, comes to mind, like, literally overnight. Right? They changed the website and we're a CDP.
And now, you know, newer vendors are trying to do the same thing. It's it's it's fascinating. The other thing, just going back to maybe more technology perspective, is when I was at Teradata and with Aster, we were 1 use case we sold was customer 360. Right? So I've been selling the notion of customer 360 since, let's say, 2007. Many enterprises, even today, still don't have a customer 360. Right? And this is, like, what? We're talking about 16 years later. Right? And it's not like they haven't done work. In my opinion, what what it is is that there's so many new customer channels and customer data sources that nobody will ever have a perfect customer 360. Right? It's always work in progress.
You're designed to connect a whole bunch of data, and by the time you do it, there's new data and new business requirements. Right? So you're always playing catch up to having the full customer 360. So you plan for a customer 3 60, but by the time you're done, you're a customer 270. And you're like, okay. Let's go build, complete it, and make it a customer 360, and then you're customer 290. Right? So you never hit the whole 360, which is why I think, enterprise solutions need to have flexibility. Right? You cannot afford to be a purist and say, I'm more than going to use data that's in this place and nothing else. Right? Because you just never know what data you're gonna need to connect and deploy in order to drive the business value that you promised to the customer.
So the in summary, nobody ever has a perfect Customer 360. There's always new data, new use cases that require different data formatting. And so as a CDP, 1 of the areas of focus we've had is to be flexible. Right? You don't wanna say, I can only use data that's in this 1 place because that may or may not be enough to deliver the promised value to the enterprise customers. You need flexibility to use the data in a central place, but also connect other data if needed. You need the flexibility to adjust the formats as needed, of course, always within the governance framework, etcetera, etcetera.
But the elusive nature of customer 360 is 1 of the things that I find very fascinating, and I always advise, technology leaders, right, to not promise that. You can make a lot of progress towards the customer 360 without getting there, and planning for having an incomplete customer 3 60 is a much more effective approach than trying to hit the full 360 in 1 go. Right? Because that's almost, like, impossible to do in a in a big enterprise environment at least.
[00:53:49] Unknown:
For people who are exploring this idea of customer data platforms or how best can I leverage the data that I have to build better experiences for my end users? What are the cases where a CDP is the wrong choice and maybe they just need to invest more in their core data capabilities or business intelligence or some other solution?
[00:54:13] Unknown:
Yeah. So so couple of things. First of all, you need some investment in in your customer infrastructure. Like, I don't think that if CDP is the first thing you're doing around customer 360, that's the wrong approach. Right? Because CDP is not a data lake. Right? CDP is is not is not Databricks. It's not Snowflake. It's not Teradata. It's not Redshift. Right? You need a database, a data lake, and you need to make some progress towards connecting some customer data. Right? Otherwise, it will be premature. I mean, it's maybe you still get some value. Right? But it won't be as much as having some infrastructure in place. Once you have some infrastructure in place, it really doesn't have to be complete or perfect. Because the CDP like Action IQ, as I mentioned before, we can be flexible, collect some data from the data lake, some data from elsewhere, and then it can evolve over time.
The second thing is I I like to focus on orchestration. Right? Are you trying is an orchestrated customer experience your goal? Because if most of what you did through 1 channel, right, if if you just want to do better email, maybe you need a better email tool. Right? Maybe you don't need a CDP, but maybe you need a better email tool. But where the CDP becomes indispensable and can provide tremendous value is the moment you need to connect different channels and different applications together, both the data and the activation, right, to to create this orchestrate experience and find the best opportunity to connect a customer. That could mean acquisition and retention, connecting together, or it could mean, you know, email, personalization, web, call center, clienteling.
Anything that that spans more than a single platform, the CDP can be extremely valuable as an orchestration tool. So this would be the 2 things I would say. Right? Have some investment in customer data on the 1 hand and then having some desire or goal for orchestration. This this make it kind of a a mature environment to deploy a CDP. And then, of course, there's a range in between. Right? But the other thing I would say is there's many different kinds of CDPs. Right? We mentioned a few before. So it's absolutely critical, you know, I think, for the whoever is doing the research to understand what are the 2 or 3 different types of CDPs, and what exactly are they looking for? And that will help them, you know, get down to, like, a couple of vendors, really. Right? And they can then explore POC to understand what fits best their needs.
[00:56:35] Unknown:
And as you continue to invest in this space of customer data platforms, improving the orchestration of information across those different channels? What are some of the things you have planned for the near to medium term at Action iQ or any particular projects or problem areas that you're excited to explore?
[00:56:54] Unknown:
Yeah. So 1 area that we're going to have some exciting announcement soon is generative AI. We've actually I I don't want to steal the thunder from our announcement, but there's 3 areas where we found tremendous value with generative AI, and and we're going to be rolling those out very soon. So the 1 that's 1 that's very exciting. You know, the the second thing is we always improve everything. Right? So if you look at our customers, we work, you know, with very large customers historically, right, a billion an app. But with a composable platform, we've invested more in making action at you composable fully self-service. Right? So if you're a data engineer, you can deploy it and manage the data and everything else completely on your own.
And that has allowed us to also attack maybe a little bit more of the middle of the market as well as the enterprise, which is our historical strength. So making Action IQ completely self-service for technical user to, you know, deploy, configure, and then hand it over to the business, to B cell service. That's another area where we've done a lot of work the past couple of years, but we're gonna be completing this work this year. I'm very excited about that as well.
[00:58:07] Unknown:
Are there any other aspects of the overall space of customer data platforms, the work you're doing at Action IQ, or the evolution of database engines and data systems that we didn't discuss yet that you'd like to cover before we close out the show?
[00:58:22] Unknown:
Now I think, you know, listen, I'm I'm excited that CDPs, I think, it's clear now that are here to stay, and I'm excited that the market is a lot more clear about who are the leaders and, you know, who are people that were just, you know, searching around for a better category. So I think you'll see tremendous growth in the CDP and and, you know, like, the 2, 3 leaders in the space over the next few years. So I think it's definitely a space to keep an eye on. It took a while to get to this point, I think, partially because it has been a confusing space about who is already in SEDP. But at this point, you know, I believe, like, in 3, 4 years, 80, 90% of enterprises will have a CDP deployed. Right? It's an absolutely necessary tool if we care about customer experience, which is what most people care about, so or most enterprises care about. So very excited about that.
[00:59:16] Unknown:
Alright. Well, for anybody who wants to get in touch with you and follow along with the work that you're doing, I'll have you add your preferred contact information to the show notes. And as the final question, I'd like to get your perspective on what you see as being the biggest gap in the tooling or technology that's available for data management today.
[00:59:32] Unknown:
I think, there's still a lot of fragmentation. You know, you would need to build a data stack. You still need to use a very large number of tools. And I think the big opportunity, especially for the data management leaders out there, is the first time someone can provide, you know, a 1 stopall that works reasonably well across all the main areas for the data management stack, I think, you know, that that company is going to be the next $1, 000, 000, 000, 000 business, because it's really hard managing all the disparate tools in the data stack. So fragmentation, I would say, is the biggest gap I see right now. But the thing with the new technologies, hardware that we're discussing earlier and and how technology is evolving, there's an opportunity to address that for the very first time, so that's that's exciting.
[01:00:21] Unknown:
Absolutely. Alright. Well, thank you very much for taking the time today to join me and share the work that you've been doing at Action IQ and the work that you have done in the database, ecosystem and your perspective of all of those different pieces and how they fit together. It's definitely been a very interesting conversation. I appreciate all the work that you're doing to help drive the ecosystem forward, and I hope you enjoy the rest of your day. I really enjoyed it. Thank you for having
[01:00:51] Unknown:
me. Thank you for listening. Don't forget to check out our other shows, podcast dot in it, which covers the Python language, its community, and the innovative ways it is being used, and the Machine Learning Podcast, which helps you go from idea to production with machine learning. Visit the site at dataengineeringpodcast.com. Subscribe to the show, sign up for the mailing list, and read the show notes. And if you've learned something or tried out a product from the show, then tell us about it. Email hosts at data engineering podcast.com with your story. And to help other people find the show, please leave a review on Apple Podcasts and tell your friends and coworkers.
Introduction to the Data Engineering Podcast
Guest Introduction: Tassos Agueros
Tassos' Background and Journey
Evolution of Database Technology
Architectural Shortcomings of Hadoop
Modern Database Technologies and Flexibility
Metadata and Data Management
Role of Customer Data Platforms (CDPs)
Composable CDPs and Organizational Impact
Governance and Data Definitions
Organizational Structure and CDP Implementation
Interesting Use Cases and Lessons Learned
When CDPs Are the Wrong Choice
Future Plans and Generative AI
Conclusion and Final Thoughts