Summary
With the rise of the web and digital business came the need to understand how customers are interacting with the products and services that are being sold. Product analytics has grown into its own category and brought with it several services with generational differences in how they approach the problem. NetSpring is a warehouse-native product analytics service that allows you to gain powerful insights into your customers and their needs by combining your event streams with the rest of your business data. In this episode Priyendra Deshwal explains how NetSpring is designed to empower your product and data teams to build and explore insights around your products in a streamlined and maintainable workflow.
Announcements
- Hello and welcome to the Data Engineering Podcast, the show about modern data management
- Join in with the event for the global data community, Data Council Austin. From March 28-30th 2023, they'll play host to hundreds of attendees, 100 top speakers, and dozens of startups that are advancing data science, engineering and AI. Data Council attendees are amazing founders, data scientists, lead engineers, CTOs, heads of data, investors and community organizers who are all working together to build the future of data. As a listener to the Data Engineering Podcast you can get a special discount of 20% off your ticket by using the promo code dataengpod20. Don't miss out on their only event this year! Visit: dataengineeringpodcast.com/data-council today!
- RudderStack helps you build a customer data platform on your warehouse or data lake. Instead of trapping data in a black box, they enable you to easily collect customer data from the entire stack and build an identity graph on your warehouse, giving you full visibility and control. Their SDKs make event streaming from any app or website easy, and their extensive library of integrations enable you to automatically send data to hundreds of downstream tools. Sign up free at dataengineeringpodcast.com/rudder
- Your host is Tobias Macey and today I'm interviewing Priyendra Deshwal about how NetSpring is using the data warehouse to deliver a more flexible and detailed view of your product analytics
Interview
- Introduction
- How did you get involved in the area of data management?
- Can you describe what NetSpring is and the story behind it?
- What are the activities that constitute "product analytics" and what are the roles/teams involved in those activities?
- When teams first come to you, what are the common challenges that they are facing and what are the solutions that they have attempted to employ?
- Can you describe some of the challenges involved in bringing product analytics into enterprise or highly regulated environments/industries?
- How does a warehouse-native approach simplify that effort?
- There are many different players (both commercial and open source) in the product analytics space. Can you share your view on the role that NetSpring plays in that ecosystem?
- How is the NetSpring platform implemented to be able to best take advantage of modern warehouse technologies and the associated data stacks?
- What are the pre-requisites for an organization's infrastructure/data maturity for being able to benefit from NetSpring?
- How have the goals and implementation of the NetSpring platform evolved from when you first started working on it?
- Can you describe the steps involved in integrating NetSpring with an organization's existing warehouse?
- What are the signals that NetSpring uses to understand the customer journeys of different organizations?
- How do you manage the variance of the data models in the warehouse while providing a consistent experience for your users?
- Given that you are a product organization, how are you using NetSpring to power NetSpring?
- What are the most interesting, innovative, or unexpected ways that you have seen NetSpring used?
- What are the most interesting, unexpected, or challenging lessons that you have learned while working on NetSpring?
- When is NetSpring the wrong choice?
- What do you have planned for the future of NetSpring?
Contact Info
Parting Question
- From your perspective, what is the biggest gap in the tooling or technology for data management today?
Closing Announcements
- Thank you for listening! Don't forget to check out our other shows. Podcast.__init__ covers the Python language, its community, and the innovative ways it is being used. The Machine Learning Podcast helps you go from idea to production with machine learning.
- Visit the site to subscribe to the show, sign up for the mailing list, and read the show notes.
- If you've learned something or tried out a project from the show then tell us about it! Email hosts@dataengineeringpodcast.com) with your story.
- To help other people find the show please leave a review on Apple Podcasts and tell your friends and co-workers
Links
- NetSpring
- ThoughtSpot
- Product Analytics
- Amplitude
- Mixpanel
- Customer Data Platform
- GDPR
- CCPA
- Segment
- Rudderstack
The intro and outro music is from The Hug by The Freak Fandango Orchestra / CC BY-SA
Sponsored By:
- TimeXtender: 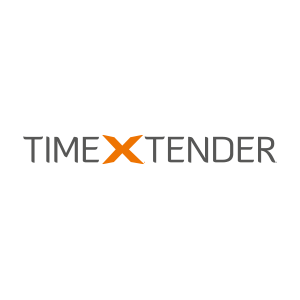 TimeXtender is a holistic, metadata-driven solution for data integration, optimized for agility. TimeXtender provides all the features you need to build a future-proof infrastructure for ingesting, transforming, modelling, and delivering clean, reliable data in the fastest, most efficient way possible. You can't optimize for everything all at once. That's why we take a holistic approach to data integration that optimises for agility instead of fragmentation. By unifying each layer of the data stack, TimeXtender empowers you to build data solutions 10x faster while reducing costs by 70%-80%. We do this for one simple reason: because time matters. Go to [dataengineeringpodcast.com/timextender](https://www.dataengineeringpodcast.com/timextender) today to get started for free!
- Rudderstack:  RudderStack provides all your customer data pipelines in one platform. You can collect, transform, and route data across your entire stack with its event streaming, ETL, and reverse ETL pipelines. RudderStack’s warehouse-first approach means it does not store sensitive information, and it allows you to leverage your existing data warehouse/data lake infrastructure to build a single source of truth for every team. RudderStack also supports real-time use cases. You can Implement RudderStack SDKs once, then automatically send events to your warehouse and 150+ business tools, and you’ll never have to worry about API changes again. Visit [dataengineeringpodcast.com/rudderstack](https://www.dataengineeringpodcast.com/rudderstack) to sign up for free today, and snag a free T-Shirt just for being a Data Engineering Podcast listener.
- Data Council: 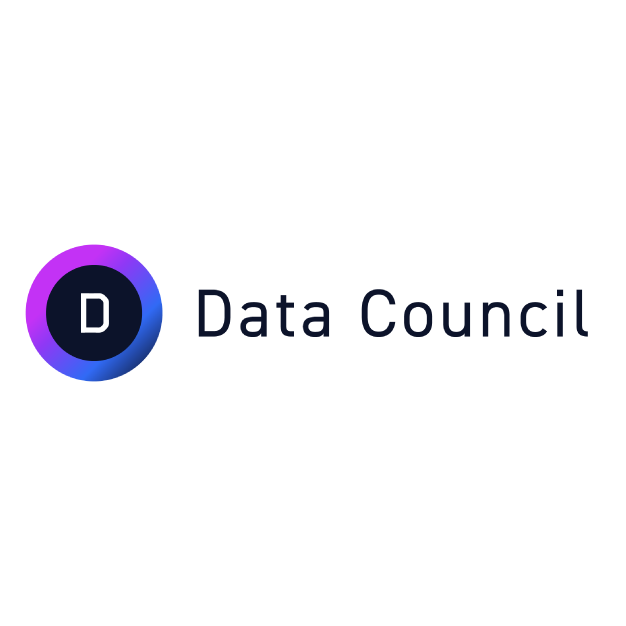 Join us at the event for the global data community, Data Council Austin. From March 28-30th 2023, we'll play host to hundreds of attendees, 100 top speakers, and dozens of startups that are advancing data science, engineering and AI. Data Council attendees are amazing founders, data scientists, lead engineers, CTOs, heads of data, investors and community organizers who are all working together to build the future of data. As a listener to the Data Engineering Podcast you can get a special discount off tickets by using the promo code dataengpod20. Don't miss out on our only event this year! Visit: [dataengineeringpodcast.com/data-council](https://www.dataengineeringpodcast.com/data-council) Promo Code: dataengpod20
Hello, and welcome to the Data Engineering Podcast, the show about modern data management. Are you tired of dealing with the headache that is the modern data stack? It's supposed to make building smarter, faster, and more flexible data infrastructure a breeze. It ends up being anything but that. Setting it up, integrating it, maintaining it, it's all kind of a nightmare. And let's not even get started on all the extra tools you have to buy to get it to work properly. But don't worry, there is a better way. Time extender takes a holistic approach to data integration that focuses on agility rather than fragmentation. By bringing all the layers of the data stack together, Time extender helps you build data solutions up to 10 times faster and saves you 70 to 80% on costs.
If you're fed up with the modern data stack, give Time extender a try. Head over to data engineering podcast.com/timeextender where you can do 2 things. Watch them build a data estate in 15 minutes and start for free today. Legacy CDPs charge you a premium to keep your data in a black box. RudderStack builds your CDP on top of your data warehouse, giving you a more secure and cost effective solution. Plus, it gives you more technical controls so you can fully unlock the power of your customer data. Visitdataengineeringpodcast.com/rudderstack today to take control of your customer data.
[00:01:30] Unknown:
Your host is Tobias Macy. And today I'm interviewing Priyendra Deshwal about how NetSpring is using the data warehouse to deliver a more flexible and detailed view of your product analytics. So, Priyendra, can you start by introducing yourself? Absolutely. Thank you so much. My name is Priyendra Deshwal. I spent, my childhood in India. My father used to work with the federal government. His job used to be moving across cities, and I had the unique experience living in 7 different cities and attending 8 different schools. Attended college in India, studied computer science at IIT Kanpur, then followed that up with a master's degree here at Stanford. And, eventually, my first job kind of landed me at Google. 1 of the things that I kind of experienced early on in coming here was, you know, this whole culture of entrepreneurship, which is very different from what I was used to at India, so that caught my eye. And so then around 2012, joined a bunch of crazy folks who were starting this business intelligence company called ThoughtSpot. Today, ThoughtSpot is hailed as a BI leader by the latest Gartner reports and valued it over $4, 000, 000, 000 in their last valuation.
While at ThoughtSpot, we kinda saw a gap where the existing products in the ecosystem did not do a great job of analyzing the time oriented, event data. And so then in 2019, we started NetSpring to solve that problem. And, working with event data since then has eventually led us to this space of warehouse native analytics that we're gonna talk about today. Thank you so much for having me, Tobias. Absolutely. And do you remember how you first got started working in data? So at Google, my first job there was with the search ranking team. And, in many ways, like, running a search engine is is all about data management. There is a lot of algorithmic aspects to it also, but then there is a lot of just managing data. So I started out pretty early on that front, but my first real exposure to enterprise data management came through, through my work at ThoughtSpot.
I got a very, close view into how enterprises are managing, storing, analyzing data,
[00:03:41] Unknown:
and and that was how I got started into it. And so in terms of what you're building at Net Spring, can you give a bit of an overview of what the focus is and some of the story behind how it came to be and why you decided that you wanted to spend your time and energy on this particular problem domain?
[00:03:57] Unknown:
Of course. Yeah. So, so our team here at Netspring has combined decades of experience of building and selling PI tools. Right? And it turns out that these products are really good at counting numbers and then dividing those numbers into categories and showing reports based on that. But there is a whole different side of analytics that involves ordering events by time. If you want to understand user retention, then you must reconstruct the user's journey through your product by laying out the event that they did, over time. And as it turns out, BI tools are really bad at it. So we started with this abstract problem of event oriented analytics.
What does it mean to understand businesses, event data that is being generated more and more now and analyze them. And, we built a pretty generic and analytically powerful system, which was very broad and could be adapted to a fair number of use cases. And it turned out that warehouse native product analytics was the killer app for this platform, and now we are going full throttle with that problem.
[00:04:59] Unknown:
And in terms of the category of product analytics, I'm wondering if you can just give a bit of an overview about the types of activities and requirements that are involved and some of the roles and teams that are going to take part in the overall exercise of collecting and creating and utilizing those product analytics?
[00:05:22] Unknown:
Of course. Of course. So the goal of product analytics is to build a deep understanding of how users are using your product and to use that understanding then to impact any number of high level metrics such as user engagement, retention, conversion, revenue, and so forth. Right? And the primary tool that these products use to understand user behavior is in product telemetry. So this telemetry, which is usually in the form of some instrumentation that you add that allows you to track events by users over time, allows you to build a very accurate history of, what a user has done in the product, and then we can use these behaviors to say, okay. I want the users to do x, but they are not doing it because they are doing by or they are failing at some earlier step that they need to cross before they can get to x.
And all of that analysis is broadly bucketed under the product analytics umbrella. The stakeholders involved are, obviously, the engineering teams are usually responsible for putting the instrumentation because the instrumentation lives inside the product. The data teams are responsible for making sure that these events and this telemetry that is produced is reliably moved from the user's browser into whatever destination. In warehouse product analytics, that destination ends up being the customer's warehouse. And, obviously, then the analyst and the product teams are responsible for mining this, repository of data for the kinds of insights that can guide product decisions and business decisions.
[00:06:54] Unknown:
And when teams first come to what you're building at NetSpring, I'm wondering what you see as some of the common challenges that they are facing and some of the ways that they have tried to address those issues prior to deciding that NetSpring is the solution that they need.
[00:07:10] Unknown:
Product analytics as a category was really invented late, around 2010. Right? That is when products like Amplitude and Mixpanel came around. These first generation products, they are built on architecture that requires customers to ship data to a third party applications. And, these companies in the decades since they have founded, they have been founded. They've done a really good job of evangelizing the need for product analytics. Today, when we walk into an account, it's not like we are trying to convince people that you need product analytics. Rather, the conversation that we are having is the problems that come with this 1st generation architecture. How can we solve them? And that is where NetSpring comes in. So the main challenges that we see are, these systems are very expensive. The part of the cost comes because it's a complete vertically integrated siloed, third party system that does everything from ingestion to storage to compute to the analytics on top. So they're quite expensive. The second concrete challenge we feel is, VCs that these systems do not have access to the entire enterprise data repository.
Typically, we see customers only send a very small fraction of their enterprise data to these third party systems. And as a result, the insights that you can generate in these systems is limited. And then finally, there is there is the underlying question of, like, poor governance. In today's world with, so much sensitivity around, data and governance, it's increasingly difficult for customers to reconcile sending such sensitive data or the wire to third party systems, and we've seen that people just want more control, of their data. So these 3 things, the cost, the access to only a fraction of the repository of data, of your enterprise data, and the the poor governance aspects of this architecture that make a solution like NetSpin attractive to these customers.
[00:09:10] Unknown:
And another interesting layer to this is the fact that you mentioned things like Mixpanel and Amplitude that are very distinctly a SaaS platform where you send all of your data to that, service. You use their instrumentation for being able to collect the different events that you're trying to track. And another, I guess, product category or at least a term that people use is the idea of a customer data platform, which platforms such as Segment helps to popularize. And I'm wondering if you can provide some distinction between this idea of customer data platform and warehouse native product analytics.
[00:09:47] Unknown:
So, I mean, 1 way to think about this is, customer data platform is kind of a broader play where you are collecting a bunch of datasets and then using it for multiple use cases. The primary 1 that most people use it for is product analytics, which is trying to understand how people are using their product, but you could also imagine experimentation, maybe testing, and other kind of workflows built on top of that same data. So in many ways, like this customer data platform plays, I mean, you can think of it as as a special purpose warehouse, which is designed around the needs of customer data. What I've seen is that this idea might have made sense a decade back when warehouse techno like, general purpose warehouse technology hadn't caught up. But in today's world, I don't see the need for a separate customer data platform. I from my perspective, the advice I would give to to people who are thinking about modern data architectures is, to put enterprise warehouse at the center of your customer data strategy and not rely on a a separate, customer data platform. And then circling back to your mention of things like data governance
[00:11:02] Unknown:
and some of the regulatory requirements around data, particularly in light of the, GDPR and CCPA legislations. I'm wondering what you see as some of the challenges involved in bringing this idea of product analytics and the collection and distribution of that information into an enterprise or highly regulated environment or industry, particularly in things like finance or health care? Yeah. So you hit the nail on the head. The main challenge
[00:11:32] Unknown:
so to so these regulatory regimes that have, come up over the last few years, they pose a big problem for any architecture that requires data to be shipped over to a different destination that is not under the control of, of your organization. Doesn't mean that people don't do it. I think the problem of product analytics is important enough that if there is no solution, people will find a way to justify it and and and get it to work. But now we have a way, and I think, increasingly
[00:12:06] Unknown:
going forward, we'll find fewer and fewer organizations opting to to send their data over. And we've been talking a little bit around this topic of the fact that there are a number of different players in this space of product analytics, both commercial and open source, and there are different generational shifts that have happened around that. And I'm wondering if you can give your perspective on the role that NetSpring plays in that ecosystem and maybe some of the cases where it serves as a replacement for a different technology, and then also the cases where it is supplemental to other offerings.
[00:12:41] Unknown:
Absolutely. So as I noted, right, so NetSprint is a warehouse native, product analytics solution, and, we deeply believe in this warehouse native, vision. So our definition of warehouse native is that no data ever leaves a customer warehouse, and no data is ever shared with the 3rd party, and no external indices are built to accelerate queries and so on and so forth. So building this kind of system under this constraint has not been easy as your perhaps familiar product analytic queries, you know, building funnels and visualizing parts of customers. They are not cheap, and, there is a lot of secret sauce to how, we are able to get such expensive workloads to perform well on on our normal data warehouse. But, in terms of ecosystem, we sit in the, we squarely sit in the analytics side of it. So we are not so we are the only truly warehouse native product on the market, and we only focus on product analytics. We are not a CDP.
We are not an instrumentation provider. We are a solution that if you have data in the warehouse, you can connect NetStream on top and very quickly start getting a valuable insights back from the system. We partner with instrumentation providers like Segment and RudderStack to, to get the data in the warehouse to begin with. Or rather our customers may use those products to, to to to get the data in the right place.
[00:14:12] Unknown:
And digging more into the platform that you've built, I'm wondering if you can talk to some of the ways that your implementation is focused on being able to best take advantage of some of the modern warehouse technologies and the associated data stacks that have grown up around those capabilities?
[00:14:31] Unknown:
Absolutely. So so our software basically flips the 1st generation product analytic architecture on its head. Right? So instead of shipping your data to an amplitude where your compute lives, NetStream will ship the compute to your data in the form of a c quickly. And the way we do that is, so I'll let me go into a little bit of the history of how we built the company. Right? So when we first started, we like, the the gap, as I noted earlier, we identified was that analyzing business data that is produced in the form of events is not a great experience, and that is the problem we started out to solve when we built an architecture that was quite generic and applicable to a broad category of use cases. And it was only once we saw traction with product analytics that we adapted that architecture to, to quote unquote warehouse native product analytics. So 1 of the first things that we did was we took everything that we knew about analytics and distilled that into novel language called NetScrip. So NetScrip, the best way to think about it is that it's an evolution of SQL that allows us to to marry the relational world of BI style analytics with the time oriented world of event oriented analytics.
So everything in next next spring is powered by NetScrip. But, of course, all of that is under the hood, and users do not need to see NetScrip directly, and they don't even need to know anything about this to to operate the system. But, ultimately, every click in the product, in the NetScrip product is backed by some kind of underlying NetScrip operation. So the 1st year and a half, we just, were building NetScrip and refining the language and getting it ready for a broad category of analytical use cases. And what we see today is that effort has paid off handsomely. Like, we have a very rigorous abstraction that sits at the heart of our system, and that abstraction allows us to do things that our other vendors are not even capable of doing. So so NetSling is not some kind of templated SQL generation, tool. It's a tool that deeply understand understands product analytics.
It can anticipate what users will do. It can precompute things for them so that, you know, results are magically available on the fly. It can optimize queries using its understanding of the underlying statistics of the data to, in many cases, be 100 of times faster than what what they would naively be. And all of that secret sauce and magic that makes warehouse native product analytics possible is ultimately the result of our investments into NetScrip.
[00:17:04] Unknown:
And when you're talking about being able to preemptively or proactively kind of pre aggregate or pre render some of the data so that it is already dimensionalized. I'm kind of reading into what you said a little bit, so that when somebody says, oh, I actually want to understand at a more granular level the exact actions that this user took when they were engaging in the product within the specific session. I'm wondering too how you think about some of the cost optimization challenges around some of these cloud native warehouses, particularly in terms of things like BigQuery and, and Snowflake, where they have different cost models, and you need to be able to be, kind of cognizant of the billing constraints that an organization might be trying to focus on and some of the ways that you are trying to optimize both for the end user experience and for the kind of financial aspects of running 1 of these systems.
[00:18:00] Unknown:
Absolutely. And, you know, this, this is 1 of the biggest questions that we get that, okay, fine. This is a compelling vision. You know? We, we are seeing some of these same problems around governance and cost, and we would like to do something, but it just seems too far fetched. Like, how can you deliver a first class product analytics experience on top of a commodity general purpose warehouse? And so the answer lies in a lot of, behind the scenes things that we have done. So, you know, the the first principle I mean, anybody building a data processing system already knows this, but the first principle is do not look at more data than you absolutely need to in order to answer a question. Right?
But the operationalizing that simple insight into practice is is, is not revealed. So the the class of techniques can go anywhere from, like, you know, simple clustering on the warehouse where you make sure that you can do as much pruning upfront to very, very advanced, pre aggregations and pre computations that, you know, maybe run once a day, but, save you, hundreds of minutes of processing time through the through the rest of the day. And each of those processing times would have build like, these warehouses are not cheap. So so there is a broad broad class of techniques that that make this kind of experience possible. The cost question is a very good 1. So big game changer with, these modern warehouses is they are built around the idea of elasticity.
So if you are using, let's say, Amplitude and you're sending a certain number of events to them, it doesn't matter. Your billing model does not take into account how much use the system is act actually getting. Like, you could do 1 query a day or you could do hundreds of queries a day, and your use your your cost will be exactly the same. Whereas with in this in this warehouse world, you are metered on how much you are actually using the system. And, by the way, we are not even in the billing path there. This customer is directly paying, the warehouse for how much use we are getting. We are only providing the analytical layer on top. And, so so the first 2 first tool to optimize cost we have in our, at our disposal in elasticity. Right? And then second tool is there is a lot of fine tuning of the size of warehouse that is required and, you know, with BigQuery, whether you need slots. And with Snowflake, what is the size of warehouse? All those things we carefully work with our customers to tune back. And then finally, it's all about, you know, working through a a specific use case. What I have seen is, there is the abstract playbook of very generic data engineering guidelines. Okay. You shard your data well. You do some sampling. All those things, they are generic. But then when once you're faced with an actual problem, you can often use your experience to craft a solution for a customer that, you know, sits within their their cost envelope.
[00:20:56] Unknown:
And another aspect of what you're building since you are operating on top of the data warehouse, is the fact that there's likely to be a high degree of variance across the specific data modeling, the level of data maturity, the types of data professionals that are within an organization. And I'm curious how you are working to simplify across those kind of dimensions of variants and be able to, operate on top of these highly customized and highly customizable systems while being able to provide a kind of consistent and cohesive experience across all of your customers?
[00:21:33] Unknown:
Yeah. It's a very good question. So there's a lot of variance. You're right. And there is organizational variance, like who does what across different companies, and then there is the data variance, which is, you know, how is data laid out. And then there is the, use case variance, which is, you know, for example, retention might mean something different for you versus some other company. Right? And so all the all those different things are there. So let's, I think it's important it's useful to start with the data variance to begin with. So 1st generation products are built with a very opinionated data model. Their data model says that you have to have a notion of the user, and you have to have a notion of, event that is generated by that user. And everything is then follows from that opinionated data model. So it works very well for your typical use case where, you know, you are trying to understand, let's say, a shopping cart and you want to see retention sorry, a funnel which shows how many people are able to successfully go through the workflow of searching for products, sending them to a cart, and checking out. So it works great for that use case. But when you come to a little bit more complicated enterprise, then it's not obvious that model is the right choice. Like, for example, consider a company like I don't know what is a good example.
Let's take, DocuSign, for example. Right? DocuSign, does it mean that I mean, there is users who are progressing through funnels, but there is also documents that are progressing through a funnel. The document is created, then they are signed by the 1st party, 2nd party, and so on and so forth, and finally, they are executed. Right? And so how do you fit something like that into the rigid data model of these first generation product? So our answer to that problem is that you don't have to fit. 1 of the benefits that we get is that we are built on top of NetScrip, which itself is built on top of SQL. So NetScrip compiles to SQL. Right? And so, therefore, we have the full richness of the underlying relational data model with all its associated joins and capabilities to join disparate datasets together. All of that richness is available to us. So we are not opinionated when it comes to what data model we are forcing on on our customers. But, obviously, it's like all of that chaos in the data model, it can't be shown to the user. Right? So what we do is we have come across, we've kind of settled across 2 concepts. 1 is the concept of an event stream, and second is the concept of an actor. An actor is something like a user, but it it could be a document. It could be anything that is going through some workflow. And event stream is the collection of events that that actor is generating over time. So in next spring, you can have, multiple event streams. You can have multiple actor types, And you can seamlessly say, okay. Show me a funnel of documents that are dropping off because 2 people are signing but the third 1 is not. Or show me the funnel of users who are able to, log on to my website and and sign up for a premium account, but then they don't use the product and churn a year later. So all those those things are possible because we have a very generic relation model at the lowest level, and, we allow users to bring their data and the product adapts to the customer's data model as opposed to the other way around.
[00:24:51] Unknown:
Join in with the event for the global data community, Data Council Austin. From March 28th to 30th 2023, they'll play host to 100 of attendees, 100 top speakers, and dozens of start ups that are advancing data science, engineering, and AI. Data Council attendees are amazing founders, data scientists, lead engineers, CTOs, heads of data, investors, and community organizers who are all working together to build the future of data. As a listener to the Data Engineering Podcast, you can get a special discount of 20% off your ticket by using the promo code data eng pod 20. Don't miss out on their only event this year. Visit data engineering podcast.com/datahyphen council today.
And another interesting aspect of being warehouse native is that the customer has to have that warehouse before they can really take make use of NetSpring. Whereas in the initial approach to product analytics, those companies were trying to be the all in 1 solution of, you don't have to have anything. Just drop this piece of JavaScript in here, and then we'll do everything for you. It's magic. And I'm wondering what your broad conception is of the kind of level of data maturity that an organization should be at to be able to make best use of NetScrip and maybe some of the ways that you have worked to ease the on ramp so that for companies who maybe haven't invested a lot in their data platform or are just starting to implement a data warehouse or able to get up to speed with being able to generate product analytics and useful insights from what NetSpring is offering without having to hire a whole suite of new data professionals or a whole new team. Yep. Absolutely.
[00:26:32] Unknown:
So the broad answer to that is that NetSpring as a product analytics solution is usable by companies across all scales. So it's not that, you know, startups cannot find value from NetScream and, and only big companies do and so forth. But that said, our observation has been that people who are feeling 1 of those 3 pains, either I already talked about this earlier. Like, either they are, troubled by the cost of operating these systems or they are troubled by the fact that only a sliver of data is accessible to product analytics, but the vast majority is not, or poor governance. If they're feeling these 3 kinds of pains or some subset of them, they are more likely to be receptive more receptive to a product like NetSuite. So what we've seen is in terms of, good fit for, working with us, if you have a warehouse and that warehouse already has events, that is a perfect starting point for us. If that is not the case, we can help you get started and we can, you know, smoothen the process of going through that journey.
But, ultimately, as I noted earlier, we are not an instrumentation company, and,
[00:27:41] Unknown:
and we we don't take ownership of that part of the problem. You've discussed this a little bit as far as some of the journey that you've gone on from the idea of NetScrip, or the the idea of NetSpring where you are today. But what are some of the other ways that the broader goals and implementation of the platform have evolved from when you first started working on it? So the goals of the platform,
[00:28:02] Unknown:
it was not like an evolution of the goals. I would frame it more as us learning what the goal should be. You know, some companies are formed with a very, very narrow kind of key product insight that okay. Okay. This, this problem needs an immediate urgent solution, and we'll, we will solve it, right away. That was not the path that NetSpring as a company took. We started broad, and we built something with the conviction that, you know, we are sure we'll find a good use case for it. Nobody has built tech like this, and, and, let's see what the market tells us. And then we dabbled in a bunch of different verticals, and we realized that this is the place to be. And, so that's that's been the path of the the company. So in terms of evolution of goals, I mean, goal has been to like, I almost view it as a as a hourglass type thing. So we start very broad in terms of the tech that we build, then we adapt that tech to a narrow use case. Right now, we are in that phase of the company. And, eventually, as we find success there, we expand again into a broader set set of use cases, and that is where the the investments that we have made in terms of building a very powerful and generic platform will actually pay off because, we'll find it much easier to expand into adjacent use cases than other products might.
[00:29:21] Unknown:
And so for teams who are adopting NetSpring and they want to be able to integrate it into their existing data warehouse, their existing data models. I'm wondering if you can talk through some of the process of getting set up with NetSpring and starting to work through that analytical process of understanding, okay, these are the data models that we have. We need to maybe add some more detail to the domain objects that we're representing within these models or add some more detail to the events that we're capturing and start to build out the informational assets that are necessary for NetSpring to be able to properly visualize and dimensionalize the different actors and events that are happening?
[00:30:03] Unknown:
Absolutely. So let me answer it for 2 class of customers separately. So there is the enterprise customer where the process is a little bit more complicated. So I come come to that later, but let's start with a small, nimble company. Literally, all we will need to get started with with with them is just, some JDBC credentials to their warehouse. And assuming that they have done the necessary instrumentation work, like, you know, integrating a library like segment or other stack into their product, we can get started right away. 1 of our product principles is 0 to wow in 1 hour, and, and we live by that. And we feel, given the right circumstances coming from the customer, we are able to deliver on that promise. What we do advise against for companies to do is to stay away from proprietary instrumentation SDKs like amplitude and mix panel because the incentives of that SDK are to put you into into their ecosystem and make it difficult to get out. So as long as you are using a a vendor neutral SDK, it's, easy enough for us to get started. Right? Now the process is fundamentally the same even at larger companies. But as you know, like, you know, with the larger transactions, there come other complications. So with the enterprise customer, we have to they usually have, like, a sprawling data lake or a warehouse, 100 to thousands of datasets.
Just navigating that environment and figuring out who has the knowledge about the use case that we, we are working on is itself a challenge. And and then we have to negotiate things like, you know, what are the datasets that next next spring should have access to? What level of access should we have? What should the size of warehouse be? And these questions are usually resolved as part of a POC process, which, takes about 2 weeks to a month or, you know, a little bit more than that.
[00:31:54] Unknown:
And as far as the types of signals that are kind of required for Net Spring to be able to operate most effectively, you mentioned that you have this concept of actors and events, and I'm wondering if you can talk to some of the common kind of data modeling activities that are necessary to be able to allow Net Spring to be able to understand what are some of the ways that we want to proactively, you know, prefetch some of this information and pre aggregate this information versus the kind of naive approach of these are the objects, but Net Spring doesn't have enough context about how they're being used or how the different actors relate to each other to be able to provide a complete view of that product.
[00:32:39] Unknown:
Absolutely. Yep. So so I'll kind of go into what is required to onboard a new customer, and I layout the journey from decision to use Next Spring to the first insight. So the steps that need to happen are we have this concept of an organization, which is basically usually 1 toward mapping with the customer. So you need to create an organization. Within our organization, there can be multiple applications. So application is a next spring concept that represents an isolated use case within a customer. So you would typically create 1 application. All of this, can be done through the UI. Then once you've created an application, within an application, you start with establishing a connection. A connection is a set of credentials to a warehouse. So, every warehouse has different, credentials.
We work with all the major ones. And, so you can create a connection, and that allows you to browse the warehouse and see what data is in there, obviously, under the con under the constraints of whatever level of access those credentials provide. Then we start this process of data mapping. So data mapping essentially says, you can see, let's say, hundreds of, datasets in the underlying warehouse. It is unlikely that on day 1, you can do something useful with all 100. Right? And our recommendation is always to start small, start with some narrow use case that is good for that that has clear business value and good proving point for the value of NetSpring to the to the customer.
So so this process of data mapping basically says, you know, you have this dataset, the stores events that are generated from, let's say, segment. So map it into the system. It's not some week long process. You can do it in minutes. And, at that point, events are, visible in next week. Then you say, okay. Where is your user's dataset? You say, okay. This is, let's map that over. So in maybe another, 30, 40 minutes or so, you have mapped all the first important, datasets that you wanted to bring into the system. And at that point, you've basically done the onboarding that you wanted. You can start now visualizing these things, using our various charting templates. You know, you can understand retention of users. You can, break up users by the kind of events that they are doing. That's typically called event segmentation in this world.
You can build funnels to understand how people are dropping off between salient points, or you can do free form browsing in the form of path analysis, which, which allows you to, see the sequence of event that people are doing. So those are the kind of main behavioral analytic, templates or reports, so to speak. Then there is the free form, because as I said earlier, we bring the best of BI and the best of the best of product analytics into into 1 place. Right? So we also have a free form exploration called visual exploration where you can just do arbitrary slice and dice in a way that, you know, I have not seen, amplitude and mixed panel support. Now they're going in that direction with some of their SQL capabilities that are being introduced. But my assessment of our free form analytics, which allow you to do arbitrary slice and dice is, is far stronger than what I've seen in other products so far.
[00:36:15] Unknown:
And given that NetSpring itself is a product organization, you're selling the product of NetSpring, and you're building a product that is aimed at organizations that are themselves building products. I'm wondering what are some of the ways that you're able to use your own platform and product to be able to help facilitate the continued evolution and implementation of the product that you're building. Of course. I mean, this is,
[00:36:39] Unknown:
this is what you would expect us to do. So we do exactly that. Instrument a product using a variety of instrumentation libraries. We have done it through snowplow, segment, rudder stack. I mean, not because we need to analyze all 3. It's that they're they're essentially the same data, but it gives us some understanding of, you know, how our customers might be using the product. Then we bring all that data into our Snowflake, and we we look at it. And this allows us this dogfooding mentality allows us to keep our ears close to the ground, and and we literally make daily improvements to our product. I mean, I truly believe that the big strength of a startup is that the round trip time between a customer and an engineer is very small, and tools like NetSpring allow us to keep it that way.
[00:37:24] Unknown:
And in your experience of building the NetSpring
[00:37:35] Unknown:
showcase the analytical breadth of, perhaps, showcase the analytical breadth of, of NetSpring. So 1 of our customers is using NetSpring to so they are a crypto company, and so their use case involves understanding user events, user behavior in the product, which is the primary use case, but then also marry those events with data that sits on the blockchain. So, so what they do is to take the data in the blockchain. They move it into their warehouse, using some kind of retail pipeline and things. So you have events and you have a transaction with blockchain and then, you know, they they do some kind of analysis that, spans these 2 these 2 categories of data. So so it's a true coming to life of our vision where we said that, not all the data is going to be in a product analytics provider, and the warehouse is the true center of gravity of enterprise data. And, and it and our recommendation is to put analytics on top of that. Another completely unrelated use case is, we have a customer that uses us for, basically, because remember, we were built as an event analytics, platform, which is much more general purpose than just product analytics. So we have a customer that uses us for understanding, events in their IoT pipeline. So there is a big manufacturing plant that sends all kinds of data or work, and they use Net Spring to generate alerts when something untoward happens or the plant is not operating according to capacity. And so it shows you the power of the underlying technology that you can take something like product analytics, and you can take something like alerting in IoT and power both of those using the same basic platform.
[00:39:27] Unknown:
And in your work and experience of building the Net Spring platform and business, what are some of the most interesting or unexpected or challenging lessons that you've learned?
[00:39:37] Unknown:
So I think I mean, for me, the whole experience around understanding how people are doing product undertakes, what is best in class in terms of instrumentation and in terms of, the kind of, rigor that, you know, these let's you look at some of these very advanced organizations who are at the forefront of using data to power their decision. They are just so good at it. And my, aspiration is to allow everybody to become that good. Like, you know, you take somebody like Airbnb and all these companies, who was of the world. They're so good at taking every single decision, consider it in isolation, and optimize it to perfection. And many companies are not able to achieve that level of flawless execution, and I would like, it's my dream to be able to enable more companies to be able to do that. So in terms of learning, I truly learned, like, what the art of the possible is in this space, and, I wanna make it accessible to as many companies as possible.
[00:40:47] Unknown:
And for people who are considering Net Spring and are facing their own challenges around product analytics or they're just getting started on that journey, what are the cases where NetSpring is the wrong choice?
[00:40:58] Unknown:
So clearly, if, you know, you're just getting started and you've not your requirements have not matured to a point where you're starting to feel these these pains around cost and data access and governance of data, then, like, it's possible to argue that, you know, maybe you're better off starting with some simpler so simpler solution. So 1 can argue maybe it's not the right choice in those circumstances. If you don't have instrumentation, then, you know, you have bigger problems. Maybe you focus on getting your instrumentation right before you, even have a conversation with us. So those are some areas that come to mind. But, honestly, I'm having a hard time answering this question because, like, it has been our explicit goal to to build NetStream in a very generic and powerful way. Right? And so I I totally believe that there is such a wide variety of use cases that NetSpring is applicable to. That if you have a question that you have some event data in your business that you wanna make sense of, This thing is probably the right tool tool for that.
[00:42:10] Unknown:
And as you continue to build and iterate on the product and explore more of the overall problem space, what are some of the things you have planned for the near to medium term or any particular projects that you're excited to dig into?
[00:42:22] Unknown:
Absolutely. So the I'll kind of answer this in various parts. 1 of the core pieces of IP that we have developed is how to run product analytics on, generic warehouses. Right? And by generic, I mean, not special purpose compute engines that are specially built for the problem of product analytics. So so we have a stake in the ground right now in terms of system that works and, is able to service things at a certain scale. But what does it mean to increase that scale, 10 x, 100 x, and so on and so forth. Right? So, really, what I want to do in the near term is to keep pushing the boundaries of the kind of scale that, NetStream is able to support.
And, so that's 1. Then the other area of immediate work for us is, you know, these our competitors have been around for decade, and, we have we are, in many ways, just starting out on this journey. And so, obviously, there is going to be product gaps in terms of the product the capabilities that, other product support versus what we do. We have we have a pretty good understanding of those gaps. We feel that we have prioritized and gotten the most important pieces done already. But there's still a set of things that we need to go down and keep meeting our competitors in terms of different kind of analysis that they can do. So that's that's number 2. Right? And then, more aspirationally, I feel that, like, in terms of where I view the space going, I feel that this distinction between BI and product analytics is very artificial.
I don't see any reason for there to be 2 different products for solving these 2 very similar problems. The set of techniques we have around event analytics can be applied to, you know, so many domains. Like, if you are analyzing customer tickets, they are the same thing. Like, you know, you create a ticket, then you assign it to somebody. Maybe the thing gets resolved, reopened. So, there are a lot of real world use cases beyond just product analytics where these event oriented analytical techniques can be very useful. Conversely, there are a lot of BI techniques like, slicing and dicing and, you know, very powerful dashboarding that are very applicable in product analytics. Like, what you'll often see is that BI tools are much more powerful, in terms of dashboarding capabilities, visualization capabilities than product analytic tools are. And there's really no reason for this divide to exist. So my vision is that, you know, in some years from now, we won't be talking about these 2 separate categories. We'll be talking about 1 powerful analytics platform, which is able to power both of these use cases. And the key problem to solve there is how do you manage all the power with the, simple UX needs of the different end users. You know, a person like a product manager is a very different persona than a a a data analyst and a data engineer. So how do you keep people with such diverse skill sets, happy in the same platform?
I have, I think, the the way forward is to is to have, like, these, idea of these templates, you know, certain templated reports, which only require a few point and click user inputs. Even people with relatively low data scale can do. And then there is the more complicated parts of, you know, authoring free form visualizations and all that perhaps only data engineers can do. So find to so, coming back to your question, like, being able to find the right balance in terms of, intuitive UX for this problem and eventually being able to offer a unified, BIM product analytics product is really, what I would like to be able to do over the next few years.
[00:46:22] Unknown:
Are there any other aspects of the NetSpring product itself or the overall space of product analytics that we didn't discuss yet that you'd like to cover before we close out the show? No. I think we had a fairly
[00:46:33] Unknown:
wide ranging discussion. I'm not able to think of any specific,
[00:46:38] Unknown:
things that we didn't touch upon. Well, for anybody who wants to get in touch with you and follow along with the work you and your team are doing, I'll have you add your preferred contact information to the show notes. And as the final question, I'd like to get your perspective on what you see as being the biggest gap in the tooling or technology that's available for data management today.
[00:46:55] Unknown:
See, we live in the era of chat gbt. Right? And, really, if you ask when the last few months have shown us, like, what is possible. And so I would say that what is possible is this super intelligent machine that's constantly watching over our data and telling us what we should know rather than us asking the question. So I feel tech has not evolved to the point where something like that is possible. I've, seen some examples of people, you know, auto generating SQL queries through chat GBT prompts, and I I can already spot errors in the SQL and so on and so forth. So I don't think we are at that point yet, but I'd And at some point, I think we will get at that point. And so the big movement that can perhaps happen is this going from pull to push based, consumption model of data. And what form that'll take? I don't know.
[00:47:56] Unknown:
Alright. Well, thank you very much for taking the time today to join me and share the work that you and your team are doing at NetSpring. It's simply a very interesting product and an interesting approach to this problem of product analytics. So it's definitely great to be seeing people, leverage the new capabilities that we have from the technologies that are out there and being able to incorporate more data sources into this very necessary and challenging problem space. So I appreciate all the time and energy that you and your team are putting into that, and I hope you enjoy the rest of your day. Absolutely. Thank you so much for having me over to, and it was a pleasure talking to you. And,
[00:48:30] Unknown:
I look forward to this coming on to the to the podcast channel.
[00:48:41] Unknown:
Thank you for listening. Don't forget to check out our other shows, podcast dot init, which covers the Python language, its community, and the innovative ways it is being used, and the Machine Learning Podcast, which helps you go from idea to production with machine learning. Visit the site at dataengineeringpodcast.com, Subscribe to the show, sign up for the mailing list, and read the show notes. And if you've learned something or tried out a product from the show, then tell us about it. Email hosts at data engineering podcast.com with your story.
And to help other people find the show, please leave a review on Apple Podcasts and tell your friends and coworkers.
Introduction and Overview of Modern Data Management
Interview with Priyendra Deshwal: Background and Career Journey
NetSpring: Focus and Evolution
Understanding Product Analytics and Stakeholders
Challenges in Product Analytics and NetSpring's Solutions
NetSpring's Role in the Product Analytics Ecosystem
Leveraging Modern Warehouse Technologies
Cost Optimization in Cloud-Native Warehouses
Handling Variance in Data Models and Organizational Structures
Data Council Austin Event Mention
Data Maturity and Onboarding with NetSpring
Evolution of NetSpring's Goals and Implementation
Setting Up and Using NetSpring for Analytics
Dogfooding NetSpring for Continuous Improvement
Customer Use Cases and Success Stories
Lessons Learned in Building NetSpring
When NetSpring Might Not Be the Right Choice
Future Plans and Aspirations for NetSpring
Closing Remarks and Contact Information