Summary
One of the most impactful technologies for data analytics in recent years has been dbt. It’s hard to have a conversation about data engineering or analysis without mentioning it. Despite its widespread adoption there are still rough edges in its workflow that cause friction for data analysts. To help simplify the adoption and management of dbt projects Nandam Karthik helped create Optimus. In this episode he shares his experiences working with organizations to adopt analytics engineering patterns and the ways that Optimus and dbt were combined to let data analysts deliver insights without the roadblocks of complex pipeline management.
Announcements
- Hello and welcome to the Data Engineering Podcast, the show about modern data management
- When you’re ready to build your next pipeline, or want to test out the projects you hear about on the show, you’ll need somewhere to deploy it, so check out our friends at Linode. With their new managed database service you can launch a production ready MySQL, Postgres, or MongoDB cluster in minutes, with automated backups, 40 Gbps connections from your application hosts, and high throughput SSDs. Go to dataengineeringpodcast.com/linode today and get a $100 credit to launch a database, create a Kubernetes cluster, or take advantage of all of their other services. And don’t forget to thank them for their continued support of this show!
- Modern data teams are dealing with a lot of complexity in their data pipelines and analytical code. Monitoring data quality, tracing incidents, and testing changes can be daunting and often takes hours to days or even weeks. By the time errors have made their way into production, it’s often too late and damage is done. Datafold built automated regression testing to help data and analytics engineers deal with data quality in their pull requests. Datafold shows how a change in SQL code affects your data, both on a statistical level and down to individual rows and values before it gets merged to production. No more shipping and praying, you can now know exactly what will change in your database! Datafold integrates with all major data warehouses as well as frameworks such as Airflow & dbt and seamlessly plugs into CI workflows. Visit dataengineeringpodcast.com/datafold today to book a demo with Datafold. RudderStack helps you build a customer data platform on your warehouse or data lake. Instead of trapping data in a black box, they enable you to easily collect customer data from the entire stack and build an identity graph on your warehouse, giving you full visibility and control. Their SDKs make event streaming from any app or website easy, and their extensive library of integrations enable you to automatically send data to hundreds of downstream tools. Sign up free at dataengineeringpodcast.com/rudder
- Data teams are increasingly under pressure to deliver. According to a recent survey by Ascend.io, 95% in fact reported being at or over capacity. With 72% of data experts reporting demands on their team going up faster than they can hire, it’s no surprise they are increasingly turning to automation. In fact, while only 3.5% report having current investments in automation, 85% of data teams plan on investing in automation in the next 12 months. 85%!!! That’s where our friends at Ascend.io come in. The Ascend Data Automation Cloud provides a unified platform for data ingestion, transformation, orchestration, and observability. Ascend users love its declarative pipelines, powerful SDK, elegant UI, and extensible plug-in architecture, as well as its support for Python, SQL, Scala, and Java. Ascend automates workloads on Snowflake, Databricks, BigQuery, and open source Spark, and can be deployed in AWS, Azure, or GCP. Go to dataengineeringpodcast.com/ascend and sign up for a free trial. If you’re a data engineering podcast listener, you get credits worth $5,000 when you become a customer.
- Your host is Tobias Macey and today I’m interviewing Nandam Karthik about his experiences building analytics projects with dbt and Optimus for his clients at Sigmoid.
Interview
- Introduction
- How did you get involved in the area of data management?
- Can you describe what Sigmoid is and the types of projects that you are involved in?
- What are some of the core challenges that your clients are facing when they start working with you?
- An ELT workflow with dbt as the transformation utility has become a popular pattern for building analytics systems. Can you share some examples of projects that you have built with this approach?
- What are some of the ways that this pattern becomes bespoke as you start exploring a project more deeply?
- What are the sharp edges/white spaces that you encountered across those projects?
- Can you describe what Optimus is?
- How does Optimus improve the user experience of teams working in dbt?
- What are some of the tactical/organizational practices that you have found most helpful when building with dbt and Optimus?
- What are the most interesting, innovative, or unexpected ways that you have seen Optimus/dbt used?
- What are the most interesting, unexpected, or challenging lessons that you have learned while working on dbt/Optimus projects?
- When is Optimus/dbt the wrong choice?
- What are your predictions for how "best practices" for analytics projects will change/evolve in the near/medium term?
Contact Info
Parting Question
- From your perspective, what is the biggest gap in the tooling or technology for data management today?
Closing Announcements
- Thank you for listening! Don’t forget to check out our other shows. Podcast.__init__ covers the Python language, its community, and the innovative ways it is being used. The Machine Learning Podcast helps you go from idea to production with machine learning.
- Visit the site to subscribe to the show, sign up for the mailing list, and read the show notes.
- If you’ve learned something or tried out a project from the show then tell us about it! Email hosts@dataengineeringpodcast.com) with your story.
- To help other people find the show please leave a review on Apple Podcasts and tell your friends and co-workers
Links
The intro and outro music is from The Hug by The Freak Fandango Orchestra / CC BY-SA
Sponsored By:
- Datafold: 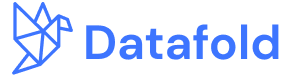 Datafold helps you deal with data quality in your pull request. It provides automated regression testing throughout your schema and pipelines so you can address quality issues before they affect production. No more shipping and praying, you can now know exactly what will change in your database ahead of time. Datafold integrates with all major data warehouses as well as frameworks such as Airflow & dbt and seamlessly plugs into CI, so in a few minutes you can get from 0 to automated testing of your analytical code. Visit our site at [dataengineeringpodcast.com/datafold](https://www.dataengineeringpodcast.com/datafold) today to book a demo with Datafold.
Hello, and welcome to the Data Engineering Podcast, the show about modern data management. When you're ready to build your next pipeline or want to test out the projects you hear about on the show, you'll need somewhere to deploy it. So check out our friends at Linode. With their new managed database service, you can launch a production ready MySQL, Postgres, or MongoDB cluster in minutes with automated backups, 40 gigabit connections from your application hosts, and high throughput SSDs. Go to data engineering podcast.com/ linode today and get a $100 credit to launch a database, create a Kubernetes cluster, or take advantage of all of their other services. And don't forget to thank them for their continued support of this show.
Modern data teams are dealing with a lot of complexity in their data pipelines and analytical code. Monitoring data quality, tracing incidents, and testing changes can be daunting and often takes hours to days or even weeks. By the time errors have made their way into production, it's often too late and the damage is done. DataFold built automated regression testing to help data and analytics engineers deal with data quality in their pull requests. DataFold shows how a change in SQL code affects your data, both on a statistical level and down to individual rows and values before it gets merged to production. No more shipping and praying. You can now know exactly what will change in your database.
DataFold integrates with all major data warehouses as well as frameworks such as Airflow and DBT and seamlessly plugs into CI workflows. Visitdataengineeringpodcast.com/datafolds today to book a demo with DataFold. Your host is Tobias Maci. And today, I'm interviewing Nandam Kartik about his experiences building analytics projects with DBT and Optimus for his clients at Sigmoid. So, Nandam, can you start by introducing yourself?
[00:01:55] Unknown:
Hi, everyone. I'm working as a senior engineering manager at Sigmoid, and I have been working in the data space for close to 4 years. And prior to that, I worked in a few gaming companies and also in b 2 b products.
[00:02:11] Unknown:
And do you remember how you first got started working in data?
[00:02:14] Unknown:
Yeah. So this happened about 4 years ago. At the time, my experience has been on the product. Around that time, I was working into data, like, gaming companies. I've also been working as a full stack in company, taking care of a couple of products end to end. So I have had the opportunity to work with a business manager, trying to understand the business requirement for the product and then take care of the end to end life cycle of delivering on that. Like, right from adding new features, deploying them to production, fixing live issues that were there on the game, and also improving performance.
So that gave me a flavor of, you know, what it feels like to work on complete product end to end. So when this opportunity came, there are a couple of things that really excited me. So 1 is, of course, the big data domain, which was new at the time. That was 1. And the other is the opportunity to lead projects end to end. So because I had a flavor of how it is when you work on projects end to end, so the scale that I understood when I work on these projects is much bigger. So that those are the 2 reasons why, you know, I got, you know, interested in the role. And that's how I got, you know, landed in the data.
[00:03:37] Unknown:
And in terms of the work that you're doing at Sigmoid, can you give a bit of background about what the company is and some of the types of projects that you're involved in?
[00:03:46] Unknown:
So Sigmoid is a data engineering and analytics company. It's act off as a product company, building analytics product engine and also a front end for it and slowly evolved into a consulting company as well. So currently, we take up analytics projects. We work on providing, building custom solutions based on the client requirements. So requirements would be from the data space. So any kind of data kind of project, we kind of, like, understand the problem and build custom solution. A few examples are cloud migration. So when there are some customers who are on prem and want to migrate to cloud. So that is 1 type of projects that we have. We also work on MLOps kind of projects. We have also worked on developing data models and productionizing them. We have also ETL pipelines end to end and also work on governance.
So different areas, like, in the data space.
[00:04:47] Unknown:
When you start to engage with some of the different clients that you work with, I'm wondering what are some of the core challenges that they're facing when they reach out to you, and if there's any sort of commonality in terms of the stage of their kind of data maturity or particular industries or geographies that you tend to work with.
[00:05:07] Unknown:
We we serve mostly North America. We have also worked with companies in South America and a few in Europe, and, of course, in India as well. So we have clients from everywhere in the world. In terms of the reasons why, you know, clients come to us is the kind of clients that come to us, some of them are early in their data journey, where they are trying to look for an expert to come in, understand the problem, and build the foundation to building their data lake. And so that down the line, a lot of analytics and, intelligence, like, AI projects can be built on top of it. Some clients have already enough maturity. They have they're already somewhere in the journey.
And they're they're looking for experts like us to come in, take up understand the problem, and deliver it quick and, you know, with the best quality. So so it's kinda, like, some little bit, you know, early in the journey where we, you know, help them a lot in terms of building it. And some clients, we offer the data engineering expertise in building solutions.
[00:06:18] Unknown:
For the conversation today, we're focusing on some of your experience of working with these clients to build out different DBT projects and then using another utility called Optimus to be able to handle the orchestration of that workflow. And in general, the overall paradigm of extract load transform with DBT being the transformation step has become fairly widespread and widely adopted particularly for analytics focused projects. And I'm wondering if you can give some examples of the types of projects that you've built with this approach and some of the types of analytics or types of questions that customers are trying to address when they engage with you and when you're working with them?
[00:07:00] Unknown:
About 1 of the projects where I have used, DBT, the company was into mining, and they have different mining sites located across the world. And at the mining sites, they have a lot of equipment and there are a lot of sensors on them which generate events, which get collected by a system. And those events are used to kind of, like, understand any kind of issues being on the site to monitor the performance of the various equipment efficiency and all. And there was an existing Excel based reporting that used to happen at every site. And what when we started on this project, what we wanted to do is make that whole process more end to end automated and also add more best practices on top of it. Because the reports are site specific. So there are reports, Excel based reports, and they are very specific to site. So there are about, like, 10, 12 sites, each site having their own, site specific format and logic for the report.
And it is also created frequently, every few weeks, manually as well. So that also introduces the, you know, error part, human error part into it. When we picked up this project, so that was the state of how the reporting was done. And and we wanted to standardize. Number 1, we wanted to automate the whole reporting and also introduce the visual layer. So So because these are all Excel based Tableau reports, like, bringing in the visual aspect to it gives a lot more, you know, understanding of the data as well. Right? It brings in a new perspective. So 1 was to automate, and the other was to visualize the data. And 3rd was to create some global reports as well. So which are more at a global level. So somebody who is sitting and wants to look at all the sites' data.
So that would basically be the global reports. So these are the 3 requirements that we had, and the events data that we were using was coming into BigQuery on Google Cloud Platform as raw data. And we have used dbt to write SQLs, which would process that data and generate tables back to BigQuery again, which are then queried to visualize the performance of basically, like, calculate different KPI metrics, which would help in analyzing the performance of the site equipment.
[00:09:37] Unknown:
As far as the overall kind of patterns or structures or project architecture that you work through, I'm wondering if there are any kind of core practices that you use as a basis across the board? And as you work with different customers, what are some of the types of changes or types of customizations that you have to add in as the particular requirements become bespoke for a given organization or use case?
[00:10:05] Unknown:
So in this particular project that I just explained, the way we were using DBT, we were using it to perform, like, transformations where we already have the data in BigQuery. So some of the patterns of how we have used TBT and also trigger these jobs on a daily basis, etcetera. This we have achieved by Dockerizing Dockerizing the code and running it on Google Cloud Engine, Google Kubernetes. And the way we were triggering them was based on schedule. So the tables that I was talking about, which was used in reporting, new data gets received every hour, and we run these jobs every year. So we have a schedule based trigger, and based on the schedule, an event gets generated. And this event is something that a process that is running as a daemon in the Kubernetes cluster picks up this event, and based on the message that is there in the event, it recognizes which flow to kind of trigger.
So accordingly, we kind of run the dbt command with some parameters which would trigger a specific flow. So the pattern here is dbt is mainly used for transmission, right, SQL and transform to create a job. And then we kind of, like, use other services on the cloud platform, listen to any triggers. The trigger trigger is scheduled. Based on the schedule, we have a mechanism, you know, to, you know, pass the event and trigger. And once the event completes again, we have a notification which can then trigger another job.
[00:11:41] Unknown:
For the dbt code, I'm wondering, as you start to work with these different customers and get them kind of up to speed on the workflow of writing DBT and building with it, what are some of the, I guess, sharp edges or white spaces in the dbt utility itself that you start to encounter and some of the ways that you've had to address some of the issues or shortcomings as you work through?
[00:12:08] Unknown:
So I have been using DBT, and DBT as a tool is also evolving. For a version of DBT, there may be some features that are not present which may be required in the project. I've encountered 1 issue that I will talk about. So in this project that I was talking about, so DBT works very well when you have a very simple, you know, requirements or simple inputs to the pipeline to run. Like, if you want so DBT allows you to so when you run DBT command, it runs for all the tables that you have as a target. Now you can, of course, choose a specific table as a target. So you can call dbt run and then the specific table name. But when you have more complex requirements, like if you want to trigger the dbt pipeline based on some inputs like start date, end date, and some kind of boolean parameters, then it won't support.
So for some versions of DBT. So at the time when I was using DBT, this feature was not available. So I had to compromise on that feature. And this requirement of passing start date, date, or any other user variables to the dbt command will be required based on your requirement. So for example, typically, when we run the pipeline, we run for previous day as an example. We get the data for previous day and we run dbt to process the data. Whenever there are any data correction that are happening to all the data, in order to fix it, you will have to run the pipeline again for the entire duration or the window of data where you have bad data which is corrected.
Now in order to fix it, we'll have to run the pipeline again. And if you don't have these kind of additional capabilities built in, like, where you can specify a start date and end date. Right? If the issue was with data for the last 10 days. Right? If you don't have this capability feature, then you'll have to run DBT, like, 10 times. In fact, that may also not work depending on how you have configured your DBT pipeline. But having this feature of providing start date and end date, where start date can be, like, 10 days before and end date can be, like, yesterday. So you can at once run for all the previous 10 days and fix the data. So when you want advanced capabilities and advanced features on your pipeline, dbt may not support. I encountered this with a version of dbt at the time. And this feature was available in the future releases.
So things to carefully look out for is whenever we are using this tool, we need to understand the use cases that we are looking at and the capabilities that we're looking at and evaluate the latest version of DBT to see that all the capabilities that you are looking to use are offered by DBT latest version. If not, then those are some of the features that you'll have to compromise.
[00:15:06] Unknown:
RudderStack helps you build a customer data platform on your warehouse or data lake. Instead of trapping data in a black box, they enable you to easily collect customer data from the entire stack and build an identity graph on your warehouse, giving you full visibility and control. Their SDKs make event streaming from any app or website easy, and their extensive library of integrations enables you to automatically send data to hundreds of downstream tools. Sign up for free today at dataengineeringpodcast dotcom/rudder.
In order to handle the orchestration of some of these projects, I know that you're using a utility called Optimus. I'm wondering if you can give a bit of overview about what that project is and some of the story behind that and the role that it plays in this dbt workflow.
[00:15:53] Unknown:
So Optimus is more of a wrapper on top of Airflow. It is a custom tool built on top of Airflow. It is more of an orchestration tool where typically when you want to build any kind of pipelines or jobs, we use Airflow connectors to build them. Now, Optimus has a wrapper on top of Airflow. Kind of, like, makes it easier and makes it configuration driven. So as an example, this particular project that I was involved. So Optimus is built based on this concept of configuration driven jobs. And when you compile this, it translates into Airflow DACHs, which get deployed in Airflow Server.
So the biggest advantage of another thing like this is it makes it easy not just for engineers to build orchestration tools and set up the jobs because it is configuration driven. And most of the jobs that we typically deal with are moving data from a table to another table. So once we have the raw data from storage, like in case of Google, so data is in Google third storage. So once you load the data into a raw BigQuery table, from there onwards, all the transformation happens on BigQuery from table to table. So majority of the transformation logic is done through SQL.
Now with tools like Optimus, which provide a plugin like bqtobq, it makes it configuration driven. So you'll have to specify a BigQuery table as a source, and you'll have to specify a target table as your destination or target, and you write your SQL. So you don't have to worry about what is Airflow, what is DAG, how to write an Airflow DAG, how to do the deployment. So it basically, you know, makes it very easy and increases the, you know, kinds of skill set people that can create jobs, production ready jobs. It's not just for engineering. It is also enabling data analysts and other, you know, experienced people to also write the jobs.
[00:18:02] Unknown:
As far as the implementation, I'm wondering if you can talk to some of the design of the Optimus tool itself and some of the ways that you thought about how to design the interfaces to allow for more of these roles to be able to interact with Typically, when we don't
[00:18:24] Unknown:
have tools like Optimus and, Typically, when we don't have tools like Optimus and if you want to productionize any kind of job like Nestle that may come from an analyst, etcetera. As a data engineer, you will have to figure out all the dependencies for the job and, you know, create airflow tags and deploy them. With tools like Optimize, a lot of it is taken care. So there is a particular pattern that we follow in terms of how we name the tables, etcetera. And when I define a job, Optimus underneath is able to identify the dependent tables automatically.
And based on the automatic detection of dependencies, it creates an airflow tag when we compile this code. So this, again, is the intelligence when you follow the patterns of Optimus and when you write a job, it automatically figures out the dependencies and deploys the Airflow tags in Airflow. Again, this design automates lot of the, you know, engineering specific workloads. Right? And allows to define the job based on config. So in addition to this job, so there are, of course, lot of CICD steps. So whenever we have any optimus job written, there are, of course, a lot of test cases that run and also converting the optimus config jobs into Airflow tag happens behind the scenes when we deploy the solution to production. The compilation happens and Airflow tags, The whole of Optimus code is compiled. And again, airflow tags are generated.
Those tags are used to replace the airflow.
[00:20:10] Unknown:
As far as the kind of tactical and organizational practices that you build up and encourage your clients to use as you're working through the implementation of these DBT projects. I'm Wondering if you can describe some of the ways that that combination of dbt and Optimus influences the ways that you think about how to structure the teams and structure the work that's being done?
[00:20:36] Unknown:
Recently, there is a trend where, you know, a lot of customers or a lot of clients, a lot of organizations are using data warehouse tools quite a lot, and this is primarily because most of these warehousing tools are becoming very capable and performant. So 3 to 4 years ago, you know, these tools were not that popular and capable, and there were other technologies that were, you know, doing the job. And recently, these tools are becoming more and more mature. We have Snowflake, which separates storage and compute. Right? So because these tools are becoming more performant and we are seeing a good adoption by organizations, SQL is becoming more of a popular, you know, scripting language to, you know, write transformations.
So it basically is enabling a lot of data analysts to write analytic based queries and easily, you know, productionize them as well. With data warehousing tools becoming more and more performant and capable, most of the organizations recently are moving towards using them more, and SQL is becoming more, predominant. Now with tools like DBT, which makes the SQL code more modular and also follow the software life cycle. It is very easy to write the code which is production ready. So prior to this prior to this kind of technology and then the tools, when we want to productionize something, there is a collaboration between different teams like business analysts and data engineers or data scientists where information exchange happens, that is a lot of knowledge transfer happens, and data engineers are responsible to production as a code. Data warehousing tools became more popular in tools like PPT and Optimus, filling lot of the automations and creating lot of these wrappers on top of typical tools, it is becoming very easy to write the production ready code at once. So definitely, there is a huge benefit in using this technology and this way of, you know, developing pipelines.
So this is some of the trend that comes in the organizations.
[00:22:57] Unknown:
For teams who are building with DBT, I'm wondering what you have found to be some of the useful kind of heuristics or strategies for understanding when dbt is the right set of tools to use to solve a particular analytical challenge and when you need to go a different route where maybe you need some more kind of custom coding or custom development or more complex transformations or pipelines to be able to achieve a particular outcome?
[00:23:28] Unknown:
When we have a typical transformation, like, simple to complex, which can be solved using SQL, we can do it with DBT. And when you have advanced processing requirements or advanced techniques to be applied where we have to use a different technology like Python or PySpark. So that's where DBT does not help. So normal data processing is something that we can do, but on top of it, if you want to run any kind of, like, Python code or any kind of, like, spark code, etcetera, you'll have to, like, you know, move away from DPT. Right? So works very well when you are doing SQL based transformations. You can use DBT to make the code more manageable and write once and easily productionize it. But when you have more advanced requirements and more complex requirements for processing data, enriching data, applying more AI technologies, DBT does not help there.
Optimus, as I said, is more of an orchestration wrapper. We can create new plugins. So we have plugins to load the data from storage to BigQuery, transfer the data from BigQuery table to BigQuery. We can even export the data from a BigQuery table to back to file system or even cloud storage. So since this is written on top of Airflow, based on the automation requirement, we can create plugins. And the advantage is you're creating the plugin once and it is configuration driven. So you're not repeating the same work again and again. You're creating a plugin. You just reuse it. And it's easy to kind of, like, again, reuse what is there and then quickly develop things and franchise.
[00:25:12] Unknown:
Data teams are increasingly under pressure to deliver. According to a recent survey by Ascend. Io, 95% reported being at or overcapacity, With 72% of data experts reporting demands on their team going up faster than they can hire, it's no surprise they are increasingly turning to automation. In fact, while only 3.5% report having current investments in automation, 85% of data teams plan on investing in automation in the next 12 months. That's where our friends at Ascend dot io come in. The Ascend Data Automation Cloud provides a unified platform for data ingestion, transformation, orchestration, and observability.
Ascend users love its declarative pipelines, powerful SDK, elegant UI, and extensible plug in architecture, as well as its support for Python, SQL, Scala, and Java. Ascend automates workloads on Snowflake, Databricks, BigQuery, and open source Spark and can be deployed in AWS, Azure, or GCP. Go to data engineering podcast dot com /ascend and sign up for a free trial. If you're a data engineering podcast listener, you get credits worth $5, 000 when you become a customer. In terms of the applications of this combination of DBT and Optimus and some of the types of projects that it's enabled you to do or some of the ways that it has empowered some of the different organizations that you've worked with. I'm wondering what are some of the most interesting or innovative or unexpected ways that you've seen them applied?
[00:26:37] Unknown:
So in DVT, in 1 of the projects, I'll give you a little bit of background about the project. It's a product with a UI where investors who wants to invest in companies would want to visit your website to kind of, like, understand about different companies. Right? So you you may want to, as an investor, you may want to compare an organization. Like, understand about the organization, understand about the revenue, and then, you know, how do you what what kind of skill set people you have in the organization, how the company has grown over the years. So you wanted to understand about a company.
And in addition, you also want to compare 2 or 3 organizations side by side and trying to compare the revenues, skill set, or different things. So to build this product, so we have USBT in a slightly interesting way. So to bring the data about the organization so that we can provide all of this information to the end user, we have been taking the LinkedIn data and that LinkedIn data is, processed and stored into different Snowflake tables. You have a jobs table. You have a revenue table. You also have, you know, employee table. You also have the issue of template. So all of the different entities, data that can come out of LinkedIn was all created from the raw data, stored it in the Snowflake tables.
So to do this transformation and processing, we have used EBT. That's 1. And whenever a user, who is typically an investor, who comes to the website, logs in, and then is searching for a company. Right? And whenever a user triggers from the UI to query about a company, the request in the back end is also automated using Airflow and using DBT. And here, the flow would basically query all the entities that were recently refreshed with the latest data from Dbt. And user specific tables are populated using the source based tables.
So this flow is also done using DBT. So 1 is to refresh the data every week from LinkedIn is 1 to keep the data latest. And based on the user commands or triggers from the UI, again, we have orchestrated the generate traditional data, specific to the user requirement using Airflow NPT and populating the user specific tables. So this is 1 of the ways that we can use DBT. It's not just for ETL. We have also used DBT to serve user requests as well from the website. It's, of course, not very quick. It takes a few minutes for the request to be served. But here in this case, that wait time is acceptable.
[00:29:28] Unknown:
And as you have been working with your customers and working with DBT some more, I'm curious if there are any strategies or approaches to how to think about structuring DBT projects or ways to kind of manage some of the iteration or specific configuration approaches that have been most useful for being able to maybe optimize build times or reduce error rates or introduce useful tests as you iterate on these projects?
[00:29:59] Unknown:
So in the DBT project that I have worked on, so we have a project template. So we have created a template for DBT project. If we need to spin off a new project using DBT, we already have the boiler template on how to use it. That is 1 pattern that we have seen that we have used successfully. The other is also, DBT allows you to write test cases. So this also helps in any time there is a coaching. DBT also allows us to write test cases and run them as part of the CICD. So this again helps in quickly iterating on any improvements that we're doing on the, DBT based products. And similarly, in Optimus as well, again, have a CICD pipeline. So whenever we have any code change and it does not meet the specifications of how we need to define jobs or the configurations are incorrect.
So every time we do a code commit, automatically, the CS50 Python kicks in and it here. So these kind of typical practices that we have followed in both the projects have been helpful, you know, using this technology and then, you know, finding out any errors.
[00:31:08] Unknown:
In your experience of working through these projects, what are some of the most interesting or unexpected or challenging lessons that you've learned in the process of combining Dbt and Optimus or helping companies to establish and iterate on their analytical approaches with these tools?
[00:31:23] Unknown:
So 1, as I said, is, of course, the version of DBT. So it's important. So 1 of the limitation that I've seen is that you'll have to evaluate the tool, look for what are your needs, and ensure that the DBT offer those capabilities. An example that I've given before. Right? The start date, make end date kind of example. And that is 1 limitation. And Optimus has the other plugin that I was talking about. So this is a plugin where which is a wrapper on Airflow, but is built for Google Cloud Platform. So if you want to use Optimus on a different cloud platform, I mean, we can take the concepts, but we have to write the plug ins again for that cloud platform. The Optimus is open source, and it has been built on Google Cloud platform. So if you are using a different cloud, then you'll have to kind of, like, extend it and put in some effort, try to manage.
That is 1 limitation, I would say. It's open source. If you're on Google Cloud, you can use it. But if you're not, then you'll have to extend the plugins. And DBT, as I said, is an evolving tool. Like, more and more features, more and more capabilities are being added. You know, before using it, we have to, you know, take it with a word of caution.
[00:32:39] Unknown:
For people who are starting to, I guess, iterate on their overall analytical approach, whether they have an existing sort of data workflow or if they're trying to build something from scratch? What are the cases where Optimus and or DBT are the wrong choice?
[00:32:58] Unknown:
DBT is wrong choice if you're not using SQL. And DBT works for some of the popular data warehousing tools, like Snowflake, Redshift, Google Cloud Platform, and a few others. If you are using a non popular warehousing tool, then dbt may not support it. So it becomes a wrong choice if if you're not with the popular data warehousing tools and not using SQL. Also, if you are using a different technology stack for your ETL pipelines for building your enterprise lake, like, for example, if you're on AWS and you're using Glue to process it, data from s 3 and write to s 3 and expose the data to Athena.
Again, changing your tech stack to use tools like Redshift and that use it could be a huge step. It, again, depends at what stage of your journey you're in on your cloud and and your data platform. If you're in early stage, you can. It depends on the type of data also that you're dealing with. Typically, for structured and semi structured data, we can load it into the warehouse and then use s twin so dbt can be used. But if you have more unstructured data, then, of course, we won't be able to use this technology.
[00:34:11] Unknown:
In terms of your kind of predictions or forward looking assessment for the evolving target of what constitutes best practices for analytics projects, I'm wondering what you see as some of the, I guess, influences that might impact change or some of the ways that the kind of evolving set of best practices is going to continue to change or shift in the near to medium term?
[00:34:38] Unknown:
A few years ago, I have seen a lot more technology options tools to process big data as we are seeing with the warehousing tools becoming more performant and capable. Tools like DBT and Optimus have come in, and they're making it easy to write in SQL and easily productionize it very quickly. With this kind of technology, it became more and more predominant, best practice expectations like traceability is 1 thing that will become common practice because everything is in tables. We are using SQL. It's easy to kind of, like, capture the lineage using tools like Informatica and others.
Few years ago, we had more technology options. So traceability was a bit of a challenge. But with everything done in data warehouse using SQL in tables, it's easy to have the leakage and the traceability. So whenever there is any data issue, it is easy to trace back and narrow it down to the origin of the issue and quickly fix it. That is 1 I see, you know, a best practice between more and more common and easy to apply. And data quality is also something that is used and applied in ETL pipelines. This, again, is becoming more and more a common best practice. And we also recommend all our clients, and we also enforce and we also apply data quality issues on the source data that comes in. We initially profile the data, and we also set up some rules to monitor the quality.
And through the ETL, once we have the process data as well, we also have some data rules that will again check the quality of the data before the data is consumed for any reporting which is used by the business to take patients and also for any other analytics case. So, again, with tools like this, you know, and, you know, data quality is also making more and more easy to apply and more common as well along with data traceability.
[00:36:46] Unknown:
Are there any other aspects of your work with DBT and Optimus and some of the ways that you're engaging with your clients to help them build out their analytical workflows with those tools that we didn't discuss yet that you'd like to cover before we close out the show? Any other base we are using, DBT and Optimus? So Optimus
[00:37:03] Unknown:
is a open source tool but built in 1 of the organizations that I'm working. There is no widespread adoption of the tool. It's currently being open source but built for specific automation requirements. DBT is, of course, a more predominant tool. And, you know, many companies, I believe, I think, 1, 000 plus companies are using DBT in production. And in terms of other ways, I think I have seen 2 ways that we have used DBT, 1 in the mining company that I spoke about, and the other is the investment product that I was talking about. So investors would like to see. So those are the 2 places that I've seen dbt use, you know, use in a in a particular way that I could work.
[00:37:44] Unknown:
Well, for anybody who wants to get in touch with you and follow with the work that you're doing, I'll have you add your preferred contact information to the show notes. And as the final question, I'd like to get your perspective on what you see as being the biggest gap in the tooling or technology that's available for data management today.
[00:37:59] Unknown:
The biggest gap is a lot of the technology and the tools that are available today creates a lot of dependency on data engineering. So that takes a lot of time and effort when you have something, some kind of, like, you know, input that comes in from different teams, data engineer becomes a bottleneck, or there is a lot of dependency on data engineering. So with tools like the bare cost tools and, DBT and optimizers tools to be more and more popular, I see, you know, less dependency on data engineering teams, making a lot more teams more capable and empowered to write SQL code, which is production driven.
So that I see as, you know, a trend going forward. Like, more automation tools where it's easy to write code And not just data engineers can productionize solutions,
[00:38:51] Unknown:
more and more teams can write directly production ready code. Alright. Well, thank you very much for taking the time today to join me and share your experiences of working with DBT and Optimus and some of the ways that this combination of tools can be used to more easily and effectively allow analytics engineers and organizations to be able to build out their different data products. So I appreciate all the time and energy that you're putting into that work and in helping to support this new open source utility. So thank you again for that, and I hope you enjoy the rest of your day. Thank
[00:39:26] Unknown:
you.
[00:39:29] Unknown:
Thank you for listening. Don't Don't forget to check out our other shows, podcast.init, which covers the Python language, its community, and the innovative ways it is being used, and the Machine Learning podcast, which helps you go from idea to production with machine learning. Visit the site at dataengineeringpodcast dotcom to subscribe to the show, sign up for the mailing list, and read the show notes. And if you've learned something or tried out a product from the show, then tell us about it. Email hosts at data engineering podcast.com with your story. And to help other people find the show, please leave a review on Apple Podcasts and tell your friends and coworkers.
Challenges in Modern Data Pipelines
Introduction to Nandam Kartik and His Journey
Overview of Sigmoid and Client Projects
Building DBT Projects for Analytics
Using Optimus for Orchestration
Structuring Teams and Workflows with DBT and Optimus
Innovative Applications of DBT and Optimus
Strategies for Structuring DBT Projects
Future Trends in Analytics Best Practices
Final Thoughts and Contact Information