Summary
Artificial intelligence technologies promise to revolutionize business and produce new sources of value. In order to make those promises a reality there is a substantial amount of strategy and investment required. Colleen Tartow has worked across all stages of the data lifecycle, and in this episode she shares her hard-earned wisdom about how to conduct an AI program for your organization.
Announcements
- Hello and welcome to the Data Engineering Podcast, the show about modern data management
- Dagster offers a new approach to building and running data platforms and data pipelines. It is an open-source, cloud-native orchestrator for the whole development lifecycle, with integrated lineage and observability, a declarative programming model, and best-in-class testability. Your team can get up and running in minutes thanks to Dagster Cloud, an enterprise-class hosted solution that offers serverless and hybrid deployments, enhanced security, and on-demand ephemeral test deployments. Go to dataengineeringpodcast.com/dagster today to get started. Your first 30 days are free!
- Data lakes are notoriously complex. For data engineers who battle to build and scale high quality data workflows on the data lake, Starburst powers petabyte-scale SQL analytics fast, at a fraction of the cost of traditional methods, so that you can meet all your data needs ranging from AI to data applications to complete analytics. Trusted by teams of all sizes, including Comcast and Doordash, Starburst is a data lake analytics platform that delivers the adaptability and flexibility a lakehouse ecosystem promises. And Starburst does all of this on an open architecture with first-class support for Apache Iceberg, Delta Lake and Hudi, so you always maintain ownership of your data. Want to see Starburst in action? Go to dataengineeringpodcast.com/starburst and get $500 in credits to try Starburst Galaxy today, the easiest and fastest way to get started using Trino.
- Join us at the top event for the global data community, Data Council Austin. From March 26-28th 2024, we'll play host to hundreds of attendees, 100 top speakers and dozens of startups that are advancing data science, engineering and AI. Data Council attendees are amazing founders, data scientists, lead engineers, CTOs, heads of data, investors and community organizers who are all working together to build the future of data and sharing their insights and learnings through deeply technical talks. As a listener to the Data Engineering Podcast you can get a special discount off regular priced and late bird tickets by using the promo code dataengpod20. Don't miss out on our only event this year! Visit dataengineeringpodcast.com/data-council and use code dataengpod20 to register today!
- Your host is Tobias Macey and today I'm interviewing Colleen Tartow about the questions to answer before and during the development of an AI program
Interview
- Introduction
- How did you get involved in the area of data management?
- When you say "AI Program", what are the organizational, technical, and strategic elements that it encompasses?
- How does the idea of an "AI Program" differ from an "AI Product"?
- What are some of the signals to watch for that indicate an objective for which AI is not a reasonable solution?
- Who needs to be involved in the process of defining and developing that program?
- What are the skills and systems that need to be in place to effectively execute on an AI program?
- "AI" has grown to be an even more overloaded term than it already was. What are some of the useful clarifying/scoping questions to address when deciding the path to deployment for different definitions of "AI"?
- Organizations can easily fall into the trap of green-lighting an AI project before they have done the work of ensuring they have the necessary data and the ability to process it. What are the steps to take to build confidence in the availability of the data?
- Even if you are sure that you can get the data, what are the implementation pitfalls that teams should be wary of while building out the data flows for powering the AI system?
- What are the key considerations for powering AI applications that are substantially different from analytical applications?
- The ecosystem for ML/AI is a rapidly moving target. What are the foundational/fundamental principles that you need to design around to allow for future flexibility?
- What are the most interesting, innovative, or unexpected ways that you have seen AI programs implemented?
- What are the most interesting, unexpected, or challenging lessons that you have learned while working on powering AI systems?
- When is AI the wrong choice?
- What do you have planned for the future of your work at VAST Data?
Contact Info
Parting Question
- From your perspective, what is the biggest gap in the tooling or technology for data management today?
Closing Announcements
- Thank you for listening! Don't forget to check out our other shows. Podcast.__init__ covers the Python language, its community, and the innovative ways it is being used. The Machine Learning Podcast helps you go from idea to production with machine learning.
- Visit the site to subscribe to the show, sign up for the mailing list, and read the show notes.
- If you've learned something or tried out a project from the show then tell us about it! Email hosts@dataengineeringpodcast.com) with your story.
Links
The intro and outro music is from The Hug by The Freak Fandango Orchestra / CC BY-SA
Sponsored By:
- Dagster: 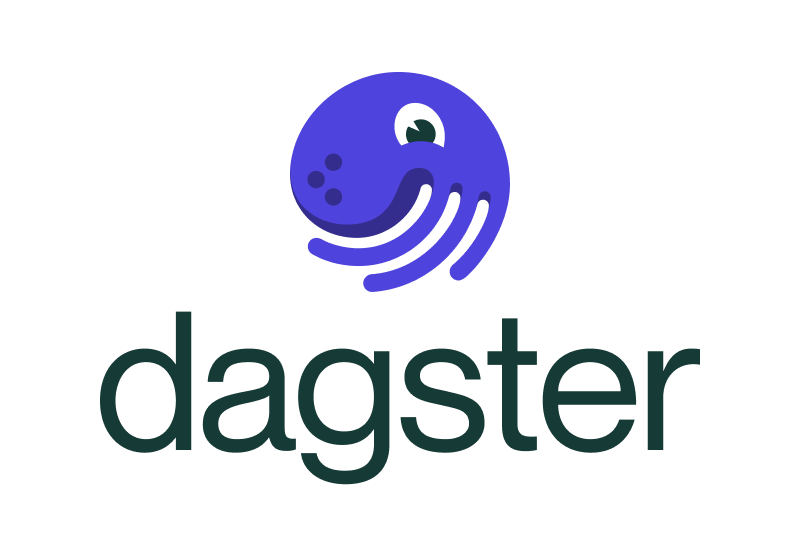 Data teams are tasked with helping organizations deliver on the premise of data, and with ML and AI maturing rapidly, expectations have never been this high. However data engineers are challenged by both technical complexity and organizational complexity, with heterogeneous technologies to adopt, multiple data disciplines converging, legacy systems to support, and costs to manage. Dagster is an open-source orchestration solution that helps data teams reign in this complexity and build data platforms that provide unparalleled observability, and testability, all while fostering collaboration across the enterprise. With enterprise-grade hosting on Dagster Cloud, you gain even more capabilities, adding cost management, security, and CI support to further boost your teams' productivity. Go to [dagster.io](https://dagster.io/lp/dagster-cloud-trial?source=data-eng-podcast) today to get your first 30 days free!
- Data Council: 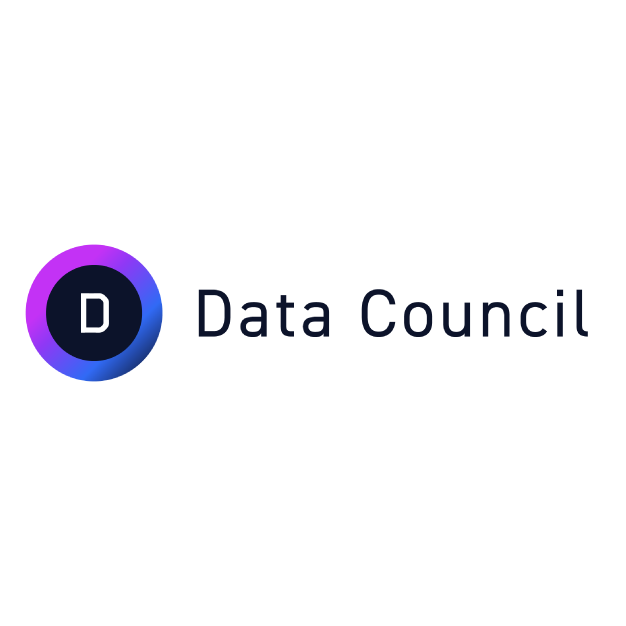 Join us at the top event for the global data community, Data Council Austin. From March 26-28th 2024, we'll play host to hundreds of attendees, 100 top speakers and dozens of startups that are advancing data science, engineering and AI. Data Council attendees are amazing founders, data scientists, lead engineers, CTOs, heads of data, investors and community organizers who are all working together to build the future of data and sharing their insights and learnings through deeply technical talks. As a listener to the Data Engineering Podcast you can get a special discount off regular priced and late bird tickets by using the promo code dataengpod20. Don't miss out on our only event this year! Visit [dataengineeringpodcast.com/data-council](https://www.dataengineeringpodcast.com/data-council) and use code **dataengpod20** to register today! Promo Code: dataengpod20
- Starburst: 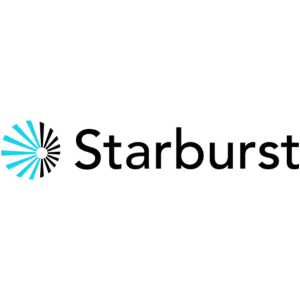 This episode is brought to you by Starburst - a data lake analytics platform for data engineers who are battling to build and scale high quality data pipelines on the data lake. Powered by Trino, Starburst runs petabyte-scale SQL analytics fast at a fraction of the cost of traditional methods, helping you meet all your data needs ranging from AI/ML workloads to data applications to complete analytics. Trusted by the teams at Comcast and Doordash, Starburst delivers the adaptability and flexibility a lakehouse ecosystem promises, while providing a single point of access for your data and all your data governance allowing you to discover, transform, govern, and secure all in one place. Starburst does all of this on an open architecture with first-class support for Apache Iceberg, Delta Lake and Hudi, so you always maintain ownership of your data. Want to see Starburst in action? Try Starburst Galaxy today, the easiest and fastest way to get started using Trino, and get $500 of credits free. [dataengineeringpodcast.com/starburst](https://www.dataengineeringpodcast.com/starburst)
Hello, and welcome to the Data Engineering podcast, the show about modern data management. Data lakes are notoriously complex. For data engineers who battle to build and scale high quality data workflows on the data lake, Starburst powers petabyte scale SQL analytics fast at a fraction of the cost of traditional methods so that you can meet all of your data needs ranging from AI to data applications to complete analytics. Trusted by teams of all sizes, including Comcast and DoorDash, Starburst is a data lake analytics platform that delivers the adaptability and flexibility a lakehouse ecosystem promises.
And Starburst does all of this on an open architecture with first class support for Apache Iceberg, Delta Lake and Hoody, so you always maintain ownership of your data. Want to see Starburst in action? Go to dataengineeringpodcast.com/starburst and and get $500 in credits to try Starburst Galaxy today, the easiest and fastest way to get started using Trino. Forms and data pipelines. It is an open source, cloud native orchestrator for the whole development lifecycle with integrated lineage and observability, a declarative programming model, and best in class testability.
Your team can get up and running in minutes thanks to Dagster Cloud, an enterprise class hosted solution that offers serverless and hybrid deployments, enhanced security, and on demand ephemeral test deployments. Go to data engineering podcast.com/daxter today to get started, and your first 30 days are free. Your host is Tobias Macy. And today, I'm bringing back Colleen Tartau to talk about the questions to answer before and during the development of an AI program. So, Colleen, can you start by introducing yourself? Yeah. Of course. Thank you, Tobias. It's great to be here again. I'm Colleen Tarto. I am field CTO and head of strategy at VAST Data.
[00:01:57] Unknown:
VAST is a company really focused on allowing our customers to activate all of their data, both structured and unstructured, in a scalable, performing, and cost efficient way. That's sort of our our holy trinity of things we're going for. So we want our customers to be able to stop stressing about managing infrastructure instead of being focusing on, getting business value out of their data. And so we're really well positioned to do this because our platform has been architected to enable BI, AI beyond. Personally, I've been in the data industry for about, oh, I don't know, over 20 years now. I've done a lot of things from data engineering to leading data and analytics programs. So I've been a user of a lot of these technologies, and I've led data science programs. So my passion is really helping make data programs successful and helping position everyone for a data driven future. And so I've, you know, been on the vendor side for a few years now.
[00:02:53] Unknown:
And for folks who haven't heard your previous appearance about a year and a half ago, do you remember how you first got involved in working in data?
[00:03:00] Unknown:
Oh, no. This is like a test I have to see if I give the same answer. Like I said, I've been in this field a long time. And during that time, there's been a lot of problems that just crop up over and over again at all of the organizations where I've worked or consulted or advised. And it's essentially it boils down to the fact that managing data is really hard. And, you know, even organizing your laptop or your Google Drive so that you can find things is really hard. So take that problem and scale it out to a multibillion dollar organization that's been collecting data for decades, and it feels like it's nearly impossible.
And I've always loved really hard problems, and I love to organize and sort through things and figure out how to categorize them to make them easier to use. And that's what data management is if you throw a lay layer of governance on top of all that, but it's a fun problem.
[00:03:47] Unknown:
For the topic today, you mentioned that we're discussing the idea of an AI program. And I'm wondering if we can try to unpack that phrase a little bit and what the organizational and technical and strategic elements are that that get involved when you say, I'm going to build an AI program.
[00:04:05] Unknown:
Yeah. That's it it is a lot packed into, 2 little words. Right? So that's a great question. And I think there's different ways you can break down any program. You can look at, like, vision, strategy, execution, or people process technology. But regardless of what you do, the vision should come first. And what I mean by that is what is the long term business goal of the program and what business need does it fill? Because AI is really cool, and every company wants to, like, change their domain to dotai and, quote, do AI. But the question is, what does that really mean? Right? It's not just your board saying we should do AI. But so you need to figure out whether there's, like, clear business value to this AI program, and that should be the first problem you solve. Because AI is super cool, but it's also really expensive, and you need to make sure the juice is worth the squeeze. And if you don't have an idea, very clear idea, of what you need from AI, then the business value won't be there and, you know, might not be successful.
And so, you know, there's a lot that comes out of that that, you know, technically obviously, there's some major major considerations as well. Do you even have the data you need to do what you wanna do? Is the data in good enough shape? Is there enough volume of the data? Because you need a lot of data to do AI. And do you have the right people? Do you have the technical expertise and infrastructure in your in your organization? Maybe. Maybe not. And it was what you wanna do even feasible. Right? You know, there's definitely a lot of research that you need to do in some capacity to answer these questions.
And so then the strategic element, you know, like, what is the actual plan? Like, once you answer enough of the questions to have an idea of what you wanna do and whether it's feasible and whether what the technology is to do it and what the people you need. You know? How are you gonna hire and execute on this? And, like, what's the timeline? What's the budget? You know? How does how do we define success? And, you know, that's the same for a lot of projects, but I think it's more paramount for AI just because it is such a such a technically rich area, and it's it's new too. Right? So a lot of these questions are open questions, and you're not gonna have all the answers, but, you know, it's not something to lightly consider. It can be a major undertaking.
[00:06:20] Unknown:
And before we get into unpacking what it even means to say AI, because there are a lot of different kind of fractal dimensions to that as well. But there's also the question of how the idea of an AI program might differ from the idea of an AI product. And, you know, it sound it sounds like 1 is a superset of the other, but I'm wondering if you could just talk through some of the ways that the difference in phrasing changes the way that you might approach the overall solution.
[00:06:46] Unknown:
Yeah. I mean, an AI product to me, like, encompasses the program, and it's really like and you're applying AI to create, like, a business solution for your for your consumers, for your end users. So, like, chat GPT is the product, but the AI program would be more like, how is it implemented, how is it maintained, etcetera. So, like, an AI program would be the technical implementation of algorithms, you know, at that scale of deep learning or machine learning and, you know, how to why does how and where does the AI code actually run? Who implements it? What's the timeline? It's much more execution focused in my mind. So the program is more like the underlying implementation and engine, whereas the product is the result.
[00:07:32] Unknown:
You mentioned that maybe AI isn't the right solution. And I'm wondering, as you are starting to embark on this process of, I want to build an AI product. This is the program go I'm going to develop to come to that outcome. What are some of the signals that you can identify early on to say, actually, this is not going to do what we think it's going to do. It's not a magic bullet. It's actually going to be 10 times more expensive than the value that it's going to produce. Just wondering what are some of the some of the questions that you should be asking early in that process to make sure that you are actually going down the path that you think you're going down.
[00:08:05] Unknown:
I mean and if we could always do that, we'd all be successful. Right? I think that answer, it's not always straightforward, and it depends like like you said, it depends what your definition of AI is. I typically am using it these days to mean deep learning and LLMs and things like that, Computer vision, you know, very complex and, like, cutting edge technologies, but it could also be, you know, a linear regression is AI to some people. So it kinda depends what you mean, but it always comes back to the question of ROI. You know, what's the expected business outcome or the value from that work or that product, and how much does it cost to implement and maintain? And that you know, that's a lot. It's like you might be really ready for this or you might not be. So I think to your question of what are some signals, I'd probably start with, is the data good enough or voluminous enough to actually do AI? Like, if you or if your day data is small, if it's not well maintained, if you don't know what you have, maybe start with that question as opposed to let's implement AI.
And then on the people side, you also need to make sure there's enough buy in and funding. Right? AI is expensive. There are ways to make it less expensive, but, you know, it's expensive. So, you know, you need to make sure that, like, as a business school, this is top tier and everyone's bought in. And then there's also, like you know, I always think about the world of health care. Are are there legal or ethical concerns? Right? So, like, they're super sensitive data in some places where, like, you can't actually it would be more challenging to implement AI because of the governance and the regulations.
So yeah. And then there's sort of 1 other question I always think back to, which is, like, do people actually want this AI? And I always go back to this the target example. I don't know if you remember this from, like I don't know. It's probably 10 or 15 years ago at this point. But, like, you know, target used machine learning to put out, like, super customized ads, and it sent ads for pregnancy products to a girl who hadn't told her parents she was pregnant yet, and her parents figured out she was pregnant from the ads or something. There was some I don't remember the exact story, but something like that. Or another example is self driving cars. Right? Like, maybe people don't want that. I don't know. Maybe they do. Maybe they don't. But, you know, you wanna make sure that
[00:10:20] Unknown:
there's actually the desire for the AI driven product before you build it. Yeah. And that the path to delivery of that AI product isn't going to be fraught with other issues. So the Yeah. The the self driving car, for instance. I think everyone can agree that they would like a car that drives itself, but they don't wanna pay the price of getting there. Yeah. I don't wanna pay the price of, you know, killing people,
[00:10:41] Unknown:
dogs, you know, possibly myself.
[00:10:44] Unknown:
Exactly. Yeah. And so for an organization that has said, okay, we think that AI is going to be an appropriate solution to this problem that we are trying to solve for. What are some of the personas that need to be involved in that process of defining the program, figuring out, do we have all of the prerequisites in place and some of the skills and systems that either need to be in place or, at least identified and requisitioned in order to be able to execute on the vision of that program.
[00:11:18] Unknown:
Yeah. I mean, the that's sort of the crux of everything. Right? Like, who and how? I think the who, the pithy answer is everyone. Right? Like, everyone should be this should be an organization wide effort. But I do think, you know, in reality, it should at the very least be a cross functional effort at the leadership level. Right? Like, leadership should own the initiative and make sure that it is a cross functional business goal and understand how to measure the success in various domains and things like that. And you also need the domain experts. You need to understand the data and the problem you're trying to solve. So if you're trying to build, I don't know, a customer support chatbot, right, you need the customer support folks to have input into the problem you're trying to solve. And, like, you know, maybe they know things that you don't know. Right? And their data engineers and data scientists aren't gonna have, like, a ton of insight into customer support necessarily. So you have to have, like, some cross functional work there with your domain experts.
And then, obviously, you need your data scientists, your data engineers, your software engineers to actually build and implement the technical solution and then also embed it in your product probably. And then you obviously need, like, testing and your stakeholder acceptance criteria, etcetera, etcetera. But, you know, I think you can make this as formal or as informal as you want, but I think testing, like, testing can go a lot of way in these things. Right? Like, I mean, the entire world is testing chat gpt. It's the most best tested product out there probably right now. But, you know, I think about in the chatbot example, there was that car dealership a few months back that had, like, an AI powered chatbot, and people were just messing with it and, you know, getting it to write programs for them and getting it to agree to sell them cars for a dollar and making it a legally binding contract and things like that. I feel like a little bit of testing probably would put a help there. So and to that end, you also need legal and compliance people to sign off on these things because, you know, again, there's regulations coming down the pipe, and there's already existing regulations about data. So something to think about. The second part of your question, I think, was the, you know, what are the skills, and I think you said the systems that need to be in place.
The execution is obviously the fun part to me. Right? Like, actually being like, okay. How do we get this data into an AI system and then have it produce a product that can be used? I mean, that's that's where the fun is. Right? But it's also where you can get lost in the weeds if you're not careful. So I think, you know, keeping an eye on, like, all of the new technology out there is a job in itself. Right? Like, there's so much out there right now. But it's gonna be the usual suspects like data engineering that are really at the crux of it. You know, they're obviously gonna be essential, but then there's also an infrastructure component, which you might not have for a BI project and an operational component that you might not have for other projects that, you know, that you're gonna need to consider for AI. So it's different than building, like, you know, a data warehouse or a b to c website. So the operation of an AI product will potentially require new skills as as well. And on the other hand, if you've already got, like, a robust data program and you've already got, like, machine learning and production, maybe it's a smaller lift because you're, like, a fairly mature data driven company. So it's kinda it means you need to, like, evaluate where you are and where you're going and sort of figure out, again, if the juice is worth the squeeze.
[00:14:37] Unknown:
Are you sick and tired of salesy data conferences? You know, the ones run by large tech companies and cloud vendors? Well, so am I. And that's why I started Data Council, the best vendor neutral, no BS data conference around. I'm Pete Soderling, and I'd like to personally invite you to Austin this March 26th to 28th, where I'll play host to hundreds of attendees, 100 plus top speakers, and dozens of hot start ups on the cutting edge of data science, engineering, and AI. The community that attends data council are some of the smartest founders, data scientists, lead engineers, CTOs, heads of data, investors, and community organizers who are all working together to build the future of data and AI.
And as a listener to the data engineering podcast, you can join us. Get a special discount off tickets by using the promo code depod20. That's depod20. I guarantee that you'll be inspired by the folks at the event, and I can't wait to see you there.
[00:15:37] Unknown:
And digging into that operational component, and you mentioned for organizations that maybe already have ML in process and, you know, exploring some of that fractal space of what is AI and, you know, a a AI has been thrown around all over the place now where, you know, it used to be expert systems, and now it's, you know, large language models that billions of parameters that get fed into them. And I'm curious, what are some of the clarifying questions that are useful in that program development phase to be able to say, actually, this doesn't even need AI. I can just throw that into, you know, an expert system, or maybe I can build some custom business logic to get the same outcome at a fraction of the expense and just some of the ways to identify what are the actual real world capabilities of AI versus just the ones that are hype and, fluff.
[00:16:33] Unknown:
Yeah. And, I mean, I think that's an excellent question. I I think we saw this, what was it, 10, 12 years ago with machine learning is everybody wanted to do machine learning. And but then when you came down to it, it was like half the problems or probably more weren't actually things that you need machine learning. You just needed some basic math and some good data, and you can answer the question. And I think, you know, I was a consultant back then, and that's I saw that time and time again. And so I think that's a big pitfall for a lot of companies is that maybe your data isn't good enough, or maybe you need to focus on this the basics before you can get into an AI ready stance.
But that said, data grows exponentially, so we're clearly gonna be in better shape now than we were 10 years ago. But it's it's still something to consider. But to your point, clearly defining that value add or, like, the problem that you're going to solve is always gonna be step 1. And I I mean, I think that's true for AI, but it's true for literally anything else a business should work on. Right? But, like, answering the questions of, like, what are you really trying to do? What does success look like? How are you gonna measure that? And then, like, once you've got those things, looking at the technology you need in order to implement it as opposed to being like, oh, this is a cool technology. Let's shove it in somewhere.
Right? Because, again, AI is expensive, and you don't wanna just it's really complex, and so you don't wanna just shove it in for the sake of shoving it and being like, we do AI. And then there's other things to consider, like, you know, maintenance and performance and regulatory compliance that it it's all a moving target and your user interactions with the product. So, like, you need to sort of figure out what does AI even mean. Right? Because it it is this amorphous term that includes anything from, like, a linear regression or, like, some basic data science all the way through deep learning and the world's most complex models and, you know, that have, like, trillions of parameters, like chat gpt or whatever. So, you know, there's sort of an incredibly broad spectrum. And so, again, focusing on what the business problem you're you're trying to solve before you try to, like, figure out what model you need, I think, is really important.
And then there's also 1 thing that came about in that sort of machine learning days of I feel it was, like, 2012 ish. 2010, 2012. But, you know, there's this trade off between complexity and clarity that's really subtle, but I think was really important back then, and it's even more important with AI because a like and meaning deep learning, because not everything is as straightforward as chat GPT. Right? Like, you know, that's part of chat gpt's beauty is its simplicity. But, you know, way back, like, 15 years ago or whatever when I was building data science program at a management consulting firm, You know, our biggest challenge was that our data scientists could do all of this, like, incredible modeling.
And the data engineering was a huge challenge, but once you got them the data, they could run for days and just, like, come up with these crazy analyses. But in the end, our clients were not ready for that. Right? They were these legacy companies that weren't equipped with the skills necessary to understand the results. So there was that huge education piece that we hadn't thought of. And so it gets back to the first question, but, like, what will AI actually deliver and is your product or your cut or are your customers ready for it? So, you know, I think there's all these different considerations that, you know, it it depends what you mean by AI and what you're what's the value you're gonna get out of it.
[00:20:06] Unknown:
And even after you've put in all the investment of time and revenue
[00:20:12] Unknown:
and energy to build the AI program, are you actually going to be able to execute on the things that it's telling you? Yeah. Absolutely. I mean, I think that's that's challenging because it could mean you have to hire a whole host of new people, or maybe you have the people. Right? But you need to know that before you invest in it. Yep.
[00:20:30] Unknown:
And and are you willing to, invest in the operational shifts that the AI is going to power and empower?
[00:20:39] Unknown:
Yeah. Absolutely. And, I mean, I think that's a piece of, like, sort of the readiness and preparation. You know? Like, are your customers gonna be okay with the new user experience even? Right? So, like, do you have this, like, basis of trust with your customers that you're then gonna be shaking? I think it's an interesting question. Yeah.
[00:21:00] Unknown:
And going back to the question of data, as you said, it can be easy to say, oh, yes. We're going to do AI, and it's going to do this thing for us, but you don't actually have any of the data that you need to be able to power that AI. And I'm curious if you can talk through some of the precursor steps and evaluations that teams can and should do to be able to build the confidence that they need, that they either have all of the data already or they can obtain all of the data and get it in shape for being able to actually power an AI.
[00:21:32] Unknown:
Yeah. I mean, I think you hit the nail on the head that defining your data needs is really step 1. Right? Like, figuring out, do you have the actual data you need? Like, if you're trying to answer a question about something, like, do you have the actual data that will help you answer that question? And so if you have it, then is it clean? Is it curated? Is it secure? Is it organized? Like, you know, if your data is lacking, you have to figure out how to get more data or better data or change what you're collecting, or maybe you need external data, maybe you can buy the data you need. And then taking that 1 step beyond, I think you need to do the same thing with your data infrastructure. And AI so AI often is working on unstructured data, and so that could be anything like video or audio or, you know, you could have semi structured data where you have some metadata that's structured and then the rest of it's unstructured. But it's not tabular data like we're used to. Right? In a BI context, it's typically tabular data or relational data.
And it turns out something like 95 percent of the world's data is unstructured, and so that's actually what AI is typically focusing on. You know, BI, on the other hand, is gonna focus on that 5% of the data structured. So from a sheer volume perspective, you're talking about a whole different game here. And so the infrastructure that you've built to handle structured data is likely not going to work for unstructured data. And so, you know, depending on what you've been doing with your unstructured data, you really need to figure out, like, how to process that because the volumes we're talking about are just completely different. Instead of talking about terabytes or gigabytes, we're talking about, you know, petabytes and exabytes. Right? And so it's just like a completely different ballgame.
So, like, I think in addition to figuring out if your data can handle AI, you also need to figure out if your data infrastructure can handle AI and what needs to be done to do that. And that sort of gets to the heart of the question about, like, data maturity. Like, you were like you were saying, there's, like, different ways to come to come through with this, but, you know, it comes down to the people, the process, the technologies, and, like, are you ready? Do you have the right people with the right skills? Do you have the right data? Do you have the right infrastructure?
And do you have an organization and a user base that's, like, data driven to a point where they're actually going to trust these results? And do they want this product? So kinda gets back to that first question. And
[00:24:01] Unknown:
because of the fact that deep learning systems and these large language models also have a lot of emergent properties, are you able to identify and apply appropriate guardrails to make sure that you're not getting
[00:24:15] Unknown:
outputs that are harmful or at least or at, you know, at worst harmful or at best confusing for the people who are interacting with it. Yeah. And I think that gets to the question of, like, really understanding what AI is doing. Like, I was explaining this to a friend of mine who's not technical, totally different world this person lives in, and, you know, they were like, I'm scared of AI. And I was like, it's just us. Right? Like, it's just taking everything we've written and writing new things that look like the old things we wrote. Right? And so I'm not scared of AI, but that said, like, I'm aware of the fact that, you know, human knowledge as a whole is biased. Right? Like, it's sexist. It's racist. It's biased. And, I mean, it's not all like that. And, you know, everything you've written, everything I've written, it's all out there. You know, the transcript from our last prod our last podcast is probably part of the data subject.
OpenAI use. Like right? It's on the Internet. And so, you know, that means that if you go and look up you know, if you ask chat gpt something, it might use our answers, which is cool. But that said, it might also use something really inappropriate, and it might create new data that then becomes part of the dataset that goes into chat GPT 5. And so, you know, it's sort of the self fulfilling prophecy in a lot of ways. It captures both the best and the worst pieces of what what's out there. And so, not to get too philosophical, but, you know, I do think that it's concerning, but like you said, there's guardrails you need to sort of think through how you're gonna put those into your product to avoid that challenge.
Yeah. So
[00:25:56] Unknown:
To to avoid selling your car for a dollar. Yeah. Exactly.
[00:26:00] Unknown:
Although that is pretty funny. It is.
[00:26:03] Unknown:
And digging more into the infrastructure and operations piece, as you mentioned, the scale and complexity of the systems required to power these data hungry and energy hungry AI models is a distinct differentiation from what people are building to power analytical products and business intelligence capabilities. And I'm wondering if you can to some of the pitfalls that teams are subject to when they say, oh, we already have a data engineering team. We already have a data platform. We can just throw AI on there. And some of the, some of the fundamental shifts that are necessary to be able to actually, operate these AI systems at scale?
[00:26:50] Unknown:
I I mean, that's that's a fantastic question. I think a lot of businesses are struggling with that right now because they're like, oh, we have a data engineering product, like you said. Like, we have a data warehouse, and it's like, well, that's great for your structured data, but what about that other 95% of your data that's unstructured that you haven't really been doing much with? And so in order to activate that data, you need to, like, kinda go back to first principles about, you know, what are your pipelines. And depending on what you're trying to do with the data, maybe you're gonna build out your own model training facility. Right? But then that's a completely different ball of wax than building a BI data warehouse. Right? Like, I I mean, it's just completely different. And so some some of the skills are gonna overlap. Some of them are very much not. And so, you know, maybe in the past, it made sense to ship your data to a cloud based system to do all your BI work, but, like, shipping the volume of data you need for AI into the cloud, I mean, that's a lot. And so maybe you wanna invest in infrastructure, or maybe you wanna look into 1 of the new the newer AI focused cloud service providers. There's a whole bunch of them out there like CoreWeave and Lambda Labs and Core 42 where their focus is to be a CSP, a cloud service provider built specifically for AI, right, as opposed to the the existing ones. So, you know, I think, you know, just because the sheer scale you're talking about and the fact that it's growing means you need to rethink everything.
And not just because, you know, cost has always been a consideration, but, you know, leaving a data warehouse running a more powerful cluster by mistake, maybe you've got a little bit of cost overrun. But, like, if you do something with AI at that scale that's wrong, like, it can cost you a lot of money. It can cost you 1, 000, 000 of dollars. So you need to be able to, like, you know, make sure that you're not doing anything like that.
[00:28:39] Unknown:
So Another element of this AI ecosystem, at least as it exists today, that is distinct from the, I guess, more predictable and sedate data platforms that are still complex in their own right is that you are inherently taking on a lot of platform risk because as somebody who doesn't have 1, 000, 000, 000 of dollars and 100 of man hours to spend on building your own custom proprietary model, you're likely going to be consuming a prebuilt model that was generated by somebody else. And so then you are subject to the update cycles of that upstream provider. Or if you're consuming it via an API, you're subject to their terms of service and pricing whims. And so I'm wondering if you can talk to some of the ways that organizations need to be considering the platform risks inherent in that, existing arrangement.
[00:29:36] Unknown:
Yeah. I mean, I think you hit the nail on the head with those few that you mentioned already. Like, I think a lot of people are challenged by the performance that they're getting out of the existing APIs and the existing models, and you're sort of, like you said, subject to the whims of those companies. And, you know, there is a risk there. And so it's like, well, is the AI work that you're doing foundational enough to your product that it makes more sense to bring something in house? And, you know, I feel like there's something new and awesome coming out to support and revolutionize the world of AI every day. Right? Like, there's so much academic research being done. There's so much research being done in some of these biggest companies. And so I think a lot of the fundamentals that we've built in the world of software and data engineering and machine learning over the years, you know, they're also going to apply in deep learning. It's just a different scale, so we need to figure out how to take what we've got and apply it to a different scale because we've always had problems with API performance.
Right? External APIs, that's always been an issue. So, you know, really understanding, like, when to bring things in house versus use something publicly available is interesting. But I do think we're at a turning point in a lot of ways where folks are starting to see the limitations of third party services too. Right? And understand that, you know, the cloud is amazing. Farming out your infrastructure is an incredible idea. You know? And who wants to hire infrastructure engineers? Right? But that said, the scales we're talking about, that might not make as much sense, and so you have to start considering alternatives. And so whether it's bringing things in house, bringing them on prem, you know, using 1 of these other technologies like an AI cloud service provider, those are considerations that we wouldn't have had to make 3 years ago. Right? So, you know, figuring out how to extend everything we've learned with our other technologies and data engineering and machine learning into, like, the deep learning and AI realm. From a process perspective, I think we actually have a lot of what we need. We just need to extend it to a different scale.
But then also, you know, the obvious question in my mind and I think a lot of people's minds is, like, what's next? Right? Because if we're rearchitecting things because we wanna take advantage of this new technology, we don't wanna be limited the next time something revolutionary comes along. Right? How do we make our business, our infrastructure, our data programs more future proof? So that's not a huge lift next time something turns everything on the way on its head the way OpenAI and chat gpt and everything have. So with that in mind, I think you need to focus on building something that really exacerbates the qualities we've always wanted in our technology stack, things like flexibility, maintainability, scalability, lack of vendor lock in, you know, easily easy governance, things like that. And so finding technologies that allow you to sort of build toward that future are really important. And then, you know, recent technologies have been coming out that have been really focusing on those things in a way that's great to see. As you mentioned,
[00:32:36] Unknown:
just about every day, there's some new product release or academic paper or, you know, acquired insight about these advanced AI and LLM systems. And so that can very quickly lead you to a sense of, uncertainty and doubt about your overall execution strategy. And I'm curious if you can talk to some of the foundational and fundamental first principles that are useful to design a platform and a program around to allow for future flexibility as this is such a constantly moving target?
[00:33:13] Unknown:
Yeah. I think, you know, over the past, I don't know how many, 20, 30, 40 years, we've, you know, been doing data when it comes to, like, you know, 20, 30 years ago. It was more about just, like, moving data and doing reporting, and then we sort of shifted into more complex BI and machine learning, and now we're doing AI. And it's like you know, I think a lot of the best practices that we've developed around, like, you know, agile project work and, you know, operational maintainability of complex online systems. Right? Like, all the DevOps work that we've done can then be applied to all of this new work, which is really cool. So I think I don't think there's a need to reinvent the wheel because this is a brand new technology because it is an evolution. It's not completely new. Right?
So I I think we can apply a lot of our best practices and our learnings from the last however many years. And, I mean, you have seen the shift in data architectures from sort of a, go with 1 vendor and stick with that vendor for 15 years. Right? And go with 1 vendor and stick with that vendor for 15 years. Right? And then we went to we went hard swing to the other way with, like, a completely composable data stack, and you've got that sort of, you know, the Matt Tirk, mad data diagram, the world of you know, there's a tool for everything. And it's it's overwhelming and it's challenging because it's not necessarily making anything easier. Now you have to maintain and manage a 1, 000 tools instead of 1 main vendor.
But, you know, that means that you can pick and choose what you want, and you could theoretically start simple. And so I think from an infrastructure and ecosystem standpoint, we're sort of hopefully landing somewhere in the middle, right, where you've got tools that work together and you've got partnerships, but then you've also got sort of huge swaths of the stack that can be handled by an individual vendor or an individual technology. And so I'm hoping that's where we're going because I do think that, you know, we've learned a lot through the last however many years. And, you know, from an infrastructure standpoint, there's so much out there that it can be really overwhelming. And if you're coupling that with, like, the most complex business problems we can think of, it's going to be it's gonna be just completely impossible to solve those problems if you're like, okay. And then we need this tool for doing this 1 thing that we then have to buy and manage and maintain. It's just the expense is gonna be crazy, and, you know, I think simplicity is really gonna be the key going forward, hopefully.
[00:35:54] Unknown:
And as you have been working in this space over the recent years and and your throughout the course of your career, I'm wondering what is 1 of the most interesting or innovative or unexpected ways that you have seen the development and execution of an AI program progress?
[00:36:11] Unknown:
I was talking to my my 9 year old the other day about this, and I was like, you know what's really cool AI? Grammar checkers. I think spell check and grammar check is amazing because it's like he doesn't need to know how to spell like we did when we were kids because it's, like, automatically changing his spelling and his grammar. But I I think, you know, 1 of the most 1 of the best things about this sort of AI revolution and what I'm looking forward to the most is seeing how scientific discovery on the scientific community responds to and uses AI. Like, I just think, you know, not just pharma, but, like, in you know, I came from academia. I came from physics. And so just, like, thinking through how that would have made my life so much easier, you know, like, having AI. And I don't just mean I would have used chat gpt to write my dissertation, but I probably would have liked to have. But, you know, I think there's a lot in the world of discovery that we can do.
And then there's the things like, you know, self driving cars and robot butlers and creative graphics and movies even that are equal parts cool and terrifying. You know? So I think, you know, we need to get over this hurdle that we're facing right now of harnessing the technology of itself. And then then we'll be able to focus on, like, sort of the human creativity aspect, and there'll be some really life changing applications of AI that'll arrive next. But I think, you know, especially in the world of pharma, you know, we had some event, and I was talking to some folks about pharma and the the things they can do with, like, personalized genomic studies that then lead to, like, personalized medications and vaccines and things like that. I mean, it's just mind blowing what's on the what's in the pipeline for that, and, obviously, there's a lot of, like, FDA hurdles to get through, but I think, you know, that's gonna it's gonna change the world. Right? Like, it's so exciting.
[00:37:58] Unknown:
In your experience of working in this space, what are some of the most interesting or unexpected or challenging lessons that you've learned personally?
[00:38:06] Unknown:
You know, this like I said, this space is fascinating, but I think 1 of the most challenging things, something to I alluded to so there's 2 things. I'm gonna give you 2 things. 1 is the data is still the problem. Right? Like, data engineering is still paramount. Right? Like, making sure you have the right data and that it's clean enough. I mean, data is never gonna be clean, but clean enough and voluminous enough and resilient enough and all those things. Like, the data engineering is still 1 of the most fun problems to me. But then also, like I said, explaining to the public what AI is.
You know, like, I, you know, I saw a person at the grocery store, and the grocery store had 1 of those robots that you can, like, ask it a simple question and it, like, mops up spills and stuff. And this woman was, like, terrified of it and ran away from it. And my kids were like, oh, that's really cool. Right? So it it's like, you know, there's definitely a generational divide. But I think most, the interesting thing is that most folks don't think about the fact that, like we said, like, ChatGPT is not a robot overlord making things up. It's literally just taking the Internet and inferring based on what's already out there. So it's based on us. And so anything we're writing and publishing is going into that dataset that's gonna feed it, and it's both amazing, and it's going to exacerbate some of our problems as a human race. So and so, like, I think people if people can understand more about it, they'll be less afraid of it, and then we'll be able to focus on the creative use of it.
Yeah. It's gonna be interesting.
[00:39:35] Unknown:
It's the linguistic version of Soylent Green. Yes. Oh my god. Yes.
[00:39:41] Unknown:
Chat GPT is people. I know. It really is. It really is. I was trying to explain that to someone, though, and they were just like, wait. So, like, if you wrote an article about something and then you ask chat gpt to write you an article about something, it would be using that. I'm like, yep. So, yeah, it's cool.
[00:40:02] Unknown:
And so for organizations that are starting down this path, they say, AI is gonna solve all of our problems. It's gonna make us all millionaires. What are the cases where AI is just absolutely the wrong choice?
[00:40:14] Unknown:
I love this question. Yeah. Because, I mean, this is like machine learning all over again. Right? And I'm gonna get back sort of to the beginning of this conversation. It's the wrong choice when you don't have the data. Right? If you don't have the data to solve the problem you're trying to solve or if you don't know what problem you're trying to solve, like, it's, again, the changing your domain to dotai and being, like, we're an AI company. It's, like, sure. But if you don't know what problem you're trying to solve and what success looks like, like, go back to the drawing board and figure that out before you start, like, looking into using an AI cloud service provider or whatever.
Because, you know, AI is I mean, I don't wanna make it sound scary because it's like something that everyone should consider, I think, but, you know, it's not always easy and it's definitely not always cheap, not yet anyway. So anyone considering an AI program, it's not always simple as, like, using the open AI API. Right? Like, that might work, but an AI program is just like any other program. You have to have, like, a clear understanding of the problem you're trying to solve and what success looks like and how it's gonna benefit your customers.
[00:41:23] Unknown:
And so as you continue to work in this space and iterate on the product that you're building at VAST Data. I'm wondering what are some of the things you have planned for the near to medium term future and some of the ways that you're looking to simplify the journey of organizations that are trying to develop and deploy their AI programs?
[00:41:42] Unknown:
Yeah. I mean, that's I'm excited about this because that's literally what we're doing at VAST. Our, our data platform is really forward thinking, and it's built to sort of help customers future proof their data program in a way that it will allow them to focus on solving the hard problems from a business perspective. Like, I mean, I think the holy grail on my mind is to get away from, you know, the intricacies of managing infrastructures and building data stacks and all this stuff and really focus on what's special to the business, which is, like, the curation of data and the, you know, using it in whatever consumption pattern makes sense. And so, you know, we're building a platform that's, you know, scalable.
We have a database so it can handle structured data. It can be you know, it's both transactional and analytical. So, you know, it can handle anything from ingest all the way through creation and consumption. And then it also handles unstructured data. We have the VAST data store, and, you know, we're just we're growing like crazy because it really does help people think toward the future. And so I've been at VAST maybe 5 or 6 months now, and, you know, we've already grown so much both in terms of business and people. And so it's been an exciting ride so far, so we'll see where it goes.
[00:42:55] Unknown:
Are there any other aspects of the promise and challenges of building and executing on an AI program that we didn't discuss yet that you'd like to cover before we close out the show?
[00:43:06] Unknown:
I mean, I think in a lot of ways, the world is our oyster. Right? Like, I I think there's so much that we can do with this that it can be daunting. And so just taking a step back and, you know, figuring out what's out there and what will benefit your business, I think, is really the the crux of the problem. But I think, you know, don't overcomplicate things. And, you know, I've been, you know, harping on this for a while, but, like, I think we need to simplify and, you know, go back to basics.
[00:43:36] Unknown:
Absolutely. Well, for anybody who wants to get in touch with you and follow along with the work that you're doing, I'll have you add your preferred contact information to the show notes. And as the final question, I'd like to get your perspective on what you see as being the biggest gap in the tooling or technology that's available for data management today. It's a really good question. I,
[00:43:54] Unknown:
you know, I think I spoke to a lot of this, but, you know, we've sort of seen this evolutionary architectural swing from monolith to fully composable stack. And then, you know, I think over time, we've done ourselves a disservice in a lot of ways because the data landscape is just, like, unmanageably complex at this point. And it's fine if you're a small start up, but, like, for larger legacy companies, it's hard to get unstuck. And so, you know, what that means in a practical and tactical sense is that the data pipelines that we've built are really, you know, fragile, and they have to be monitored and managed and governed, and they're intricate and hard to manage. And so the problems that we're trying to solve have also gotten more complex and challenging.
And so, like I just said, like, I think we need to go back to a simpler stack with fewer pipelines. Like, the more you move around data, the harder it's gonna be to get the answers you need. So fewer pipelines, less complexity, and focus on the value of the data. Like, every customer, I always am like, what are you trying to do? Like, what business problem are you trying to solve? Like, ignore our software. Ignore your infrastructure. Like, what are you trying to do? And And it always gets back to that question. So I think, you know, that's what we need to do as a as a data society.
[00:45:10] Unknown:
Alright. Well, thank you very much for taking the time today to join me and share your perspective on how to build and execute on an AI program and some of the pitfalls to be aware of in the early and mid to late stages of that process. So, definitely appreciate the time and energy that you and your team are putting into making that an easier problem to tackle, and I hope you enjoy the rest of your day. Thank you so much for having me. It's been great being back here with you, Tobias, and looking forward to seeing where everything goes.
[00:45:44] Unknown:
Thank you for listening. Don't forget to check out our other shows, podcast.init, which covers the Python language, its community, and the innovative ways it is being used, and the Machine Learning podcast, which helps you go from idea to production with machine learning. Visit the site at dataengineeringpodcast dotcom. Subscribe to the show, sign up for the mailing list, and read the show notes. And if you've learned something or tried out a product from the show, then tell us about it. Email hosts at data engineering podcast.com with your story.
And to help other people find the show, please leave a review on Apple Podcasts and tell your friends and coworkers.
Introduction and Overview
Guest Introduction: Colleen Tartau
Challenges in Data Management
Building an AI Program: Vision and Strategy
AI Program vs. AI Product
Evaluating AI Feasibility
Key Personas in AI Program Development
Operational Components of AI
Clarifying AI Needs and Capabilities
Data Readiness for AI
Guardrails and Ethical Considerations
Infrastructure and Operations for AI
Platform Risks in AI
Foundational Principles for AI Programs
Innovative AI Applications
Lessons Learned in AI Development
When AI is the Wrong Choice
Future Plans at VAST Data
Final Thoughts and Closing